Solar energy collection power prediction method based on grey neural network
A gray neural network and solar energy collection technology, which is applied in the field of solar energy collection power prediction based on gray neural network, can solve the problem of high model prediction error, achieve the effect of solving small sample prediction problems, improving prediction accuracy and generalization ability
- Summary
- Abstract
- Description
- Claims
- Application Information
AI Technical Summary
Problems solved by technology
Method used
Image
Examples
Embodiment Construction
[0038] The present invention will be further described below in combination with specific embodiments.
[0039] Such as figure 1 Said, the present invention provides a kind of gray neural network based solar energy collection power prediction method,
[0040] (1) Collect and organize solar energy data
[0041] Collect and count solar energy, temperature, humidity, and wind speed data, and take solar energy S, temperature T, humidity H, and wind speed data W at m time every day in n days.
[0042] (2) Establish GM(1,N)
[0043] Based on the 3 factors affecting solar energy and solar energy collected in step (1), there are n groups in total, and a gray prediction model with temperature T, humidity H, and wind speed W as factor variables and solar energy S as behavioral variables is established. Specific steps are as follows:
[0044] ① order As the original training sequence, the accumulation of the above sequences generates a 1-AGO sequence recorded as
[0045] ②The ...
PUM
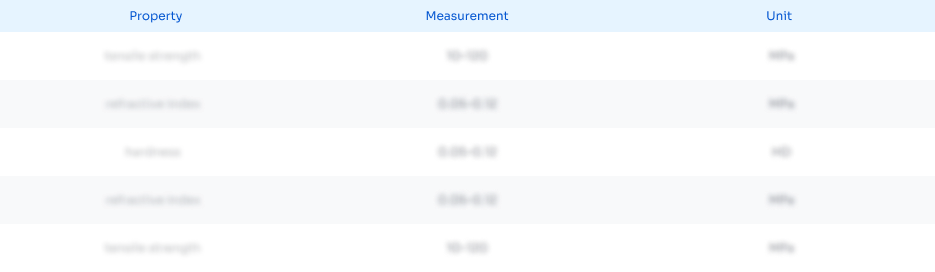
Abstract
Description
Claims
Application Information
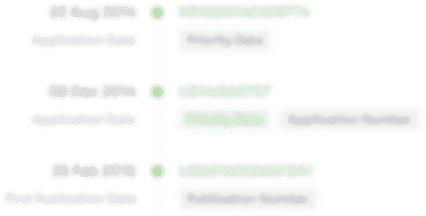
- R&D Engineer
- R&D Manager
- IP Professional
- Industry Leading Data Capabilities
- Powerful AI technology
- Patent DNA Extraction
Browse by: Latest US Patents, China's latest patents, Technical Efficacy Thesaurus, Application Domain, Technology Topic, Popular Technical Reports.
© 2024 PatSnap. All rights reserved.Legal|Privacy policy|Modern Slavery Act Transparency Statement|Sitemap|About US| Contact US: help@patsnap.com