Differential evolution algorithm with variable crossover probability factor
A differential evolution algorithm and cross probability factor technology, applied in genetic models, genetic laws, etc., can solve problems such as differences and performance differences, and achieve the effect of reducing the number of iterations, computing time, and memory consumption.
- Summary
- Abstract
- Description
- Claims
- Application Information
AI Technical Summary
Problems solved by technology
Method used
Image
Examples
Embodiment 1
[0078] Embodiment 1: This example compares the efficiency of two differential evolution algorithm cross factor determination schemes in the time modulation array antenna pattern optimization, one is the scheme proposed by the present invention, and the other is the traditional fixed cross factor scheme. The case compares which scheme requires fewer iterations to reduce the fitness value of the population below the threshold. In the case of a fixed objective function, the number of iterations required reflects the efficiency of numerical optimization.
[0079] Here, the optimized vector length J is 36, and the number of populations is N P The value is 4.0 times J, and the variation factor F is 0.6. In the traditional scheme, the crossover factor CR is set to 0.9. In the scheme proposed by the present invention, the low-end parameter CR of the crossover probability low and the high-end parameter CR up The values are 0.1 and 0.9 respectively.
[0080] When the total number ...
Embodiment 2
[0081] Example 2: This example compares the efficiency of two differential evolution algorithm crossover factor determination schemes in the optimization of the time modulation array antenna pattern, one is the scheme proposed by the present invention, and the other is the linear increase in crossover probability in the literature Program:
[0082]
[0083] where CR min and CR max are the minimum and maximum values of the crossover probability, g is the number of optimization iterations, and G is the total number of iterations of optimization iterations. The crossover probability in this scheme increases linearly with the number of iterations, from CR min increases all the way to CR max .
[0084] Here, the optimized vector length J is 36, and the number of populations is N P The value is 4.0 times J, and the variation factor F is 0.6. In the scheme proposed by the present invention, the low-end parameter CR of the crossover probability low and the high-end paramet...
PUM
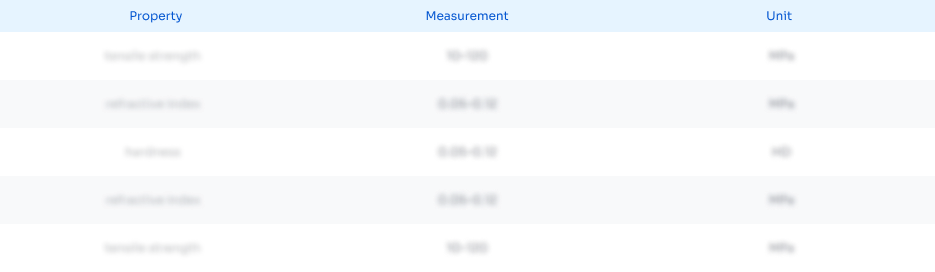
Abstract
Description
Claims
Application Information
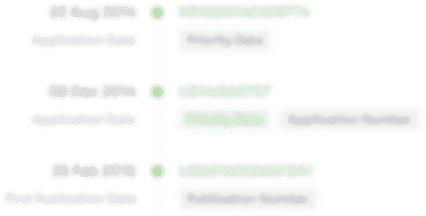
- R&D Engineer
- R&D Manager
- IP Professional
- Industry Leading Data Capabilities
- Powerful AI technology
- Patent DNA Extraction
Browse by: Latest US Patents, China's latest patents, Technical Efficacy Thesaurus, Application Domain, Technology Topic, Popular Technical Reports.
© 2024 PatSnap. All rights reserved.Legal|Privacy policy|Modern Slavery Act Transparency Statement|Sitemap|About US| Contact US: help@patsnap.com