Local model migration learning-based gear fault recognition method
A technology of transfer learning and fault identification, applied in character and pattern recognition, computer parts, instruments, etc., can solve problems such as difficulty in diagnosing variable speed and environmental conditions, reducing fault identification accuracy, and difficulty in meeting diagnostic needs.
- Summary
- Abstract
- Description
- Claims
- Application Information
AI Technical Summary
Problems solved by technology
Method used
Image
Examples
Embodiment Construction
[0082] The present invention will be described in detail below in conjunction with the accompanying drawings and specific embodiments.
[0083] Such as figure 1 As shown, a gear fault identification method based on local model transfer learning, this method can fully mine a large number of auxiliary samples that are similar to the target sample in the case of a small amount of target sample data to help the fault identification of the target data, Including the following steps:
[0084] (1) The time-frequency domain feature extraction is performed on the gear under the target specific task to obtain the target data feature vector A = [ε 1 , ε 2 ,...,ε 59 ], the same feature extraction is performed on the gear under the non-target specific task to obtain the auxiliary data feature vector B j =[δ (j,1) ,δ (j,2) ,...,δ (j,59) ]. Among them, A is the 59-dimensional target feature vector, ε i is the extracted time-frequency domain feature, the subscript i∈{1, 2,..., 59} is...
PUM
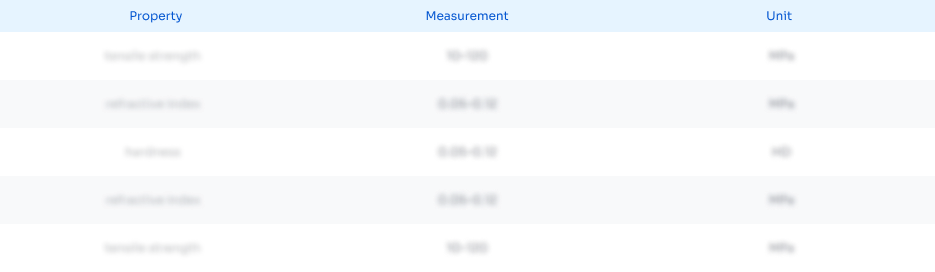
Abstract
Description
Claims
Application Information
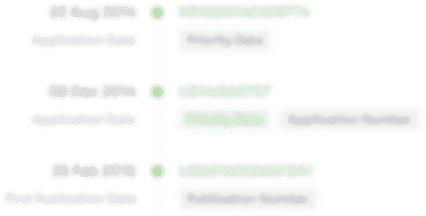
- Generate Ideas
- Intellectual Property
- Life Sciences
- Materials
- Tech Scout
- Unparalleled Data Quality
- Higher Quality Content
- 60% Fewer Hallucinations
Browse by: Latest US Patents, China's latest patents, Technical Efficacy Thesaurus, Application Domain, Technology Topic, Popular Technical Reports.
© 2025 PatSnap. All rights reserved.Legal|Privacy policy|Modern Slavery Act Transparency Statement|Sitemap|About US| Contact US: help@patsnap.com