A Deep Bottleneck Feature Extraction Method of Cardiac Shock Signal Based on Deep Neural Network
A deep neural network and cardiac shock signal technology, applied in neural learning methods, biological neural network models, neural architectures, etc., can solve problems such as being susceptible to external environment interference, and achieve improved cardiac function representation performance and high robustness. , the effect of overcoming dependence
- Summary
- Abstract
- Description
- Claims
- Application Information
AI Technical Summary
Problems solved by technology
Method used
Image
Examples
Embodiment Construction
[0044] Detailed description of the specific embodiments of the present invention will be described in conjunction with the drawings.
[0045] A method of extracting a deep bottleneck characteristic of a cardioptric impact signal based on a deep neural network,
[0046] Step 1: Determine the input vector and target vector form of the neural network: synchronously detect the electrocardiographic signal ECG signal and heart impact signal BCG signal of the same subject, and preprocessing the two, and acquires the input vector of the depth neural network. Target vector.
[0047] Step 1.1: Collect the synchronous ECG signal, BCG signal of the same subject, and normalize it separately;
[0048] Step 1.2: Get the R wave position of the ECG signal, the J wave position of the BCG signal, and separate the ECG, the BCG signal separately, and uniformly acquire 70 sample points as one frame (the signal sample rate is 100 Hz);
[0049] Step 1.3: Determine the input vector: Each frame BCG signal,...
PUM
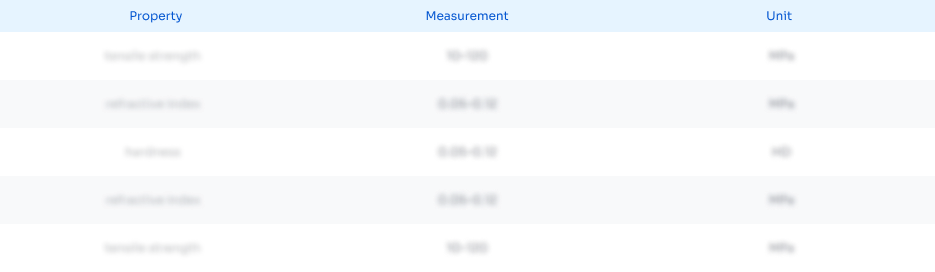
Abstract
Description
Claims
Application Information
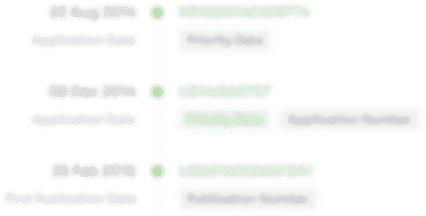
- R&D Engineer
- R&D Manager
- IP Professional
- Industry Leading Data Capabilities
- Powerful AI technology
- Patent DNA Extraction
Browse by: Latest US Patents, China's latest patents, Technical Efficacy Thesaurus, Application Domain, Technology Topic, Popular Technical Reports.
© 2024 PatSnap. All rights reserved.Legal|Privacy policy|Modern Slavery Act Transparency Statement|Sitemap|About US| Contact US: help@patsnap.com