Image classification method based on semi-supervised self-paced learning cross-task deep network
A deep network and classification method technology, applied in the field of computer vision, can solve problems such as network overfitting and inability to handle large-scale data sets
- Summary
- Abstract
- Description
- Claims
- Application Information
AI Technical Summary
Problems solved by technology
Method used
Image
Examples
Embodiment
[0079] This embodiment provides an image classification method based on semi-supervised self-paced learning cross-task depth network, the flow chart of the method is as follows figure 1 shown, including the following steps:
[0080] S1. Randomly select a small number of labeled samples from the overall image data set, and keep their labels. All the remaining samples are regarded as unlabeled samples. Their real labels are not known throughout the process. The weight of labeled samples is always 1 during the training process. The weight of the unlabeled sample is initialized to 0. Initially, only the labeled sample is used as the training set, and the samples in the training set are further expanded, and 4 pixels of zero are added around the image for padding, and then a random image of the size of the original image is taken. image;
[0081] S2. Using the training set to train the cross-task deep network, the structural diagram of the cross-task deep network is as follows i...
PUM
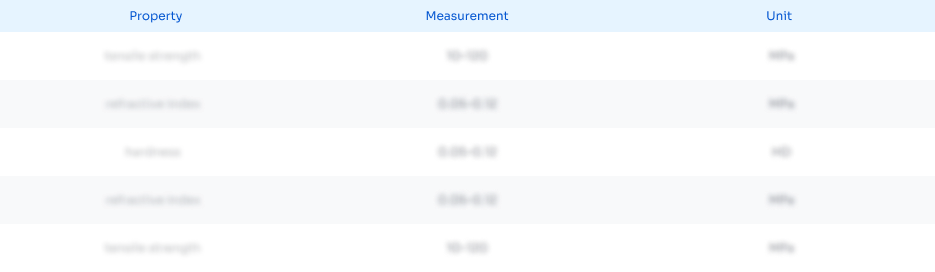
Abstract
Description
Claims
Application Information
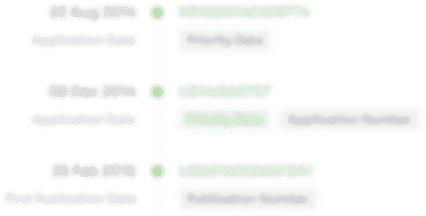
- R&D
- Intellectual Property
- Life Sciences
- Materials
- Tech Scout
- Unparalleled Data Quality
- Higher Quality Content
- 60% Fewer Hallucinations
Browse by: Latest US Patents, China's latest patents, Technical Efficacy Thesaurus, Application Domain, Technology Topic, Popular Technical Reports.
© 2025 PatSnap. All rights reserved.Legal|Privacy policy|Modern Slavery Act Transparency Statement|Sitemap|About US| Contact US: help@patsnap.com