Heart sound classification method and system based on CNN combined with improved frequency wavelet slice transformation
A classification method and heart sound technology, applied in neural learning methods, instruments, biological neural network models, etc., can solve problems such as large differences in heart sound signals, inability to achieve classification, and multiple interference signals in heart sound signals, and achieve improved accuracy and good performance. The effect of classification ability
- Summary
- Abstract
- Description
- Claims
- Application Information
AI Technical Summary
Problems solved by technology
Method used
Image
Examples
Embodiment 1
[0057] Such as figure 1 As shown, Embodiment 1 of the present disclosure provides a heart sound classification method based on CNN combined with improved frequency wavelet slice transform, divides the data into two parts according to the sample entropy value, and trains two different parameters on the two parts of data respectively. Convolutional Neural Network (Convolutional Neural Network) models. When performing ten-fold cross-validation, different convolutional neural network models (model 1 and model 2 ) to classify, the heart sound signal greater than the sample entropy threshold adopts the model 1 For classification, the heart sound signal smaller than the sample entropy threshold adopts the model 2 Classification results show that this method has good classification ability for heart sound signals.
[0058] Specifically include the following aspects:
[0059] (1) Data selection
[0060] The present embodiment adopts the public database of the Heart Sound Challeng...
Embodiment 2
[0140] Embodiment 2 of the present disclosure provides a heart sound classification system combining CNN with improved frequency wavelet slice transformation, including:
[0141] The preprocessing module is configured to: preprocess the acquired heart sound signal, use the hidden semi-Markov model to find the position of each cardiac cycle signal of each heart sound signal, intercept each cardiac cycle signal, and find the first cardiac cycle signal. sound, second heart sound, systole, and diastole;
[0142] The data conversion module is configured to: utilize the improved frequency slice wavelet transform to convert each intercepted one-dimensional cardiac cycle signal into a two-dimensional time-frequency image;
[0143] The network training and classification module is configured to: respectively calculate the sample entropy of the acquired heart sound signal, and compare it with the preset sample entropy threshold; when the sample entropy of the heart sound signal is great...
Embodiment 3
[0145] Embodiment 3 of the present disclosure provides a medium on which a program is stored, and when the program is executed by a processor, the steps in the heart sound classification method of CNN combined with improved frequency wavelet slice transformation as described in Embodiment 1 of the present disclosure are realized.
PUM
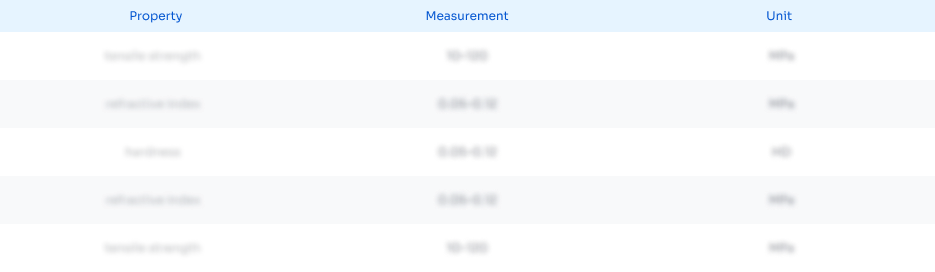
Abstract
Description
Claims
Application Information
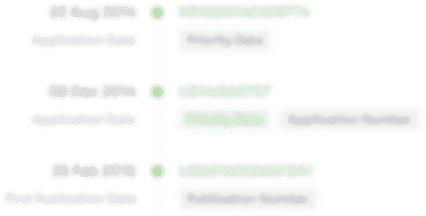
- R&D Engineer
- R&D Manager
- IP Professional
- Industry Leading Data Capabilities
- Powerful AI technology
- Patent DNA Extraction
Browse by: Latest US Patents, China's latest patents, Technical Efficacy Thesaurus, Application Domain, Technology Topic, Popular Technical Reports.
© 2024 PatSnap. All rights reserved.Legal|Privacy policy|Modern Slavery Act Transparency Statement|Sitemap|About US| Contact US: help@patsnap.com