Supervised learning optimization method under tensor mode and system thereof
A technology of supervised learning and optimization methods, applied in the field of supervised learning optimization methods and systems, which can solve problems such as local minimum, high algorithm time complexity, and dimension disaster
- Summary
- Abstract
- Description
- Claims
- Application Information
AI Technical Summary
Problems solved by technology
Method used
Image
Examples
Embodiment 1
[0077] figure 1 The implementation process of the supervised learning optimization method in the tensor mode provided by Embodiment 1 of the present invention is shown, and the details are as follows:
[0078] In step S101, an input training tensor dataset is received.
[0079] In the embodiment of the present invention, it is assumed that the training tensor data set is {Xm,ym|m=1,2.....M}, where X m Represents tensor input data, y m ∈{+1,-1} denotes a label.
[0080] Taking a grayscale image as an example, the sample points are stored in the form of a second-order tensor (matrix), and all sample points form the input data set in the form of a column vector. Similarly, the label set is also a column vector, and the value of each label The position corresponds to the position of the corresponding sample point.
[0081]
[0082] In step S102, the intra-class scatter matrix is introduced into the objective function, so that the objective function maximizes the inter-cla...
Embodiment 2
[0139] figure 2 A specific structural block diagram of a supervised learning optimization system in tensor mode provided by Embodiment 2 of the present invention is shown. For convenience of description, only parts related to the embodiment of the present invention are shown. The supervised learning optimization system 2 under the tensor mode includes: a data receiving unit 21, an intra-class distribution introduction unit 22, a sub-problem optimization framework construction unit 23, a problem optimization framework construction unit 24, a dual problem acquisition unit 25, and a dual problem solving unit unit 26 , projection tensor calculation unit 27 , projection tensor decomposition unit 28 , back projection unit 29 , optimal projection tensor calculation unit 210 , decision function construction unit 211 and prediction unit 212 .
[0140] Wherein, the data receiving unit 21 is used to receive the input training tensor data set;
[0141] Intra-class dispersal introduction...
PUM
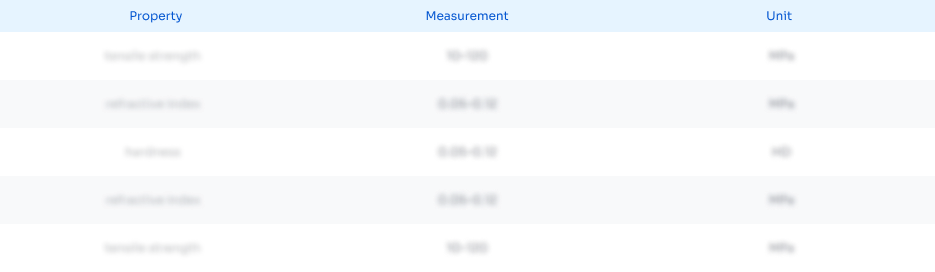
Abstract
Description
Claims
Application Information
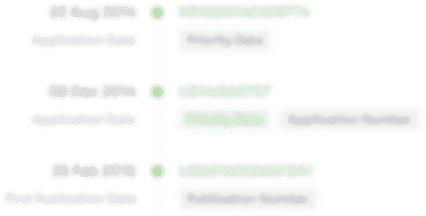
- Generate Ideas
- Intellectual Property
- Life Sciences
- Materials
- Tech Scout
- Unparalleled Data Quality
- Higher Quality Content
- 60% Fewer Hallucinations
Browse by: Latest US Patents, China's latest patents, Technical Efficacy Thesaurus, Application Domain, Technology Topic, Popular Technical Reports.
© 2025 PatSnap. All rights reserved.Legal|Privacy policy|Modern Slavery Act Transparency Statement|Sitemap|About US| Contact US: help@patsnap.com