Short-time traffic flow prediction method based on spatial-temporal correlation
A short-term traffic flow, time-space correlation technology, applied in traffic flow detection, road vehicle traffic control system, forecasting, etc., can solve the problem of increasing the complexity of the model, not considering the characteristics of traffic flow, and difficult to reflect the complex characteristics of traffic flow data and other issues to achieve the effect of improving the prediction accuracy
- Summary
- Abstract
- Description
- Claims
- Application Information
AI Technical Summary
Problems solved by technology
Method used
Image
Examples
Embodiment 1
[0072] The short-term traffic flow prediction method based on spatio-temporal correlation in this embodiment, the main flow chart and its structure diagram are as follows figure 1 with figure 2 shown, including the following steps:
[0073] Step 1: Select the road section that needs to be predicted for traffic flow and the breakpoints in the road section, and obtain the short-term traffic flow historical data of all breakpoints in the selected road section;
[0074] Step 2, according to the obtained short-term traffic flow historical data, determine the prediction period of short-term traffic flow prediction;
[0075] Step 3, according to the short-term traffic flow historical data of the breakpoint, verify whether the historical traffic flow data of the predicted breakpoint is periodic;
[0076] Step 4, using the normalization method to normalize the traffic flow data, and divide the normalized data set into a training data set and a testing data set;
[0077] Step five, ...
PUM
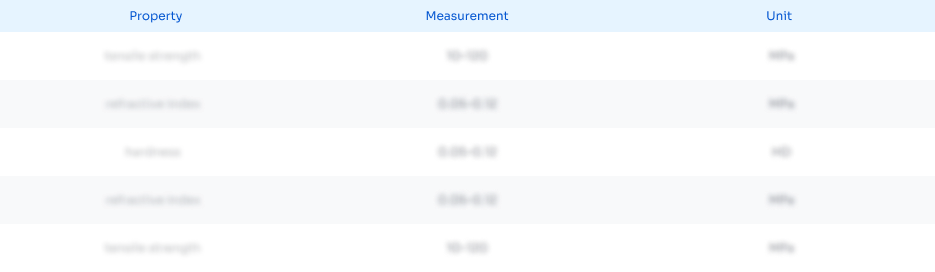
Abstract
Description
Claims
Application Information
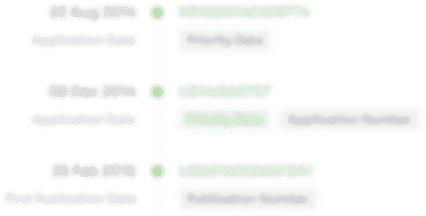
- Generate Ideas
- Intellectual Property
- Life Sciences
- Materials
- Tech Scout
- Unparalleled Data Quality
- Higher Quality Content
- 60% Fewer Hallucinations
Browse by: Latest US Patents, China's latest patents, Technical Efficacy Thesaurus, Application Domain, Technology Topic, Popular Technical Reports.
© 2025 PatSnap. All rights reserved.Legal|Privacy policy|Modern Slavery Act Transparency Statement|Sitemap|About US| Contact US: help@patsnap.com