Deep self-coding neural network-based electroencephalogram EEG signal feature extraction method
A neural network and EEG technology, applied in the field of EEG feature extraction based on deep self-encoding neural network, can solve the problems of lack of objectivity and scientificity, ignoring the mutual influence of scalp electrodes, etc. The effect of small input dimensions
- Summary
- Abstract
- Description
- Claims
- Application Information
AI Technical Summary
Problems solved by technology
Method used
Image
Examples
Embodiment Construction
[0050] The present invention will be further described in detail below in conjunction with the embodiments and the accompanying drawings, but the embodiments of the present invention are not limited thereto.
[0051] Such as Figure 1~4 Shown, a kind of EEG feature extraction method based on deep self-encoding neural network, comprises the following steps:
[0052] Step 1, design the EEG data acquisition experimental scheme for color recognition;
[0053] Design a cycle with three test pictures and three all-black transition pictures. The time for the test picture is t1, and the time for the transition picture is t2. The test pictures in each cycle are the three primary colors of red, green, and blue, and the three primary colors of red, green, and blue appear The sequence is random, so one cycle takes 3t1+3t2, and each subject tests N cycles, sharing time N(3t1+3t2); the purpose of setting transition pictures is to eliminate the visual residue generated when switching test p...
PUM
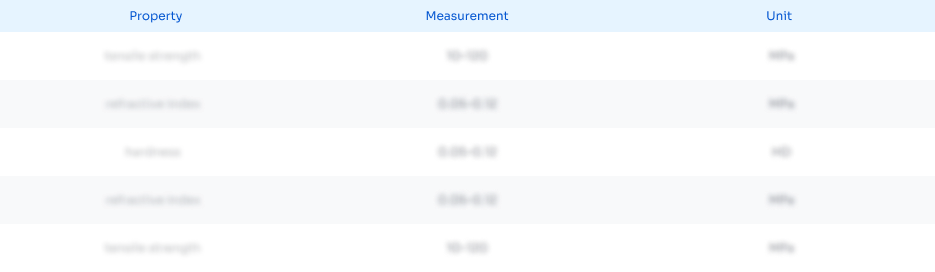
Abstract
Description
Claims
Application Information
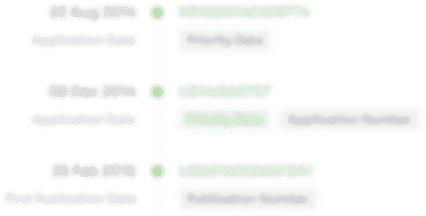
- R&D Engineer
- R&D Manager
- IP Professional
- Industry Leading Data Capabilities
- Powerful AI technology
- Patent DNA Extraction
Browse by: Latest US Patents, China's latest patents, Technical Efficacy Thesaurus, Application Domain, Technology Topic, Popular Technical Reports.
© 2024 PatSnap. All rights reserved.Legal|Privacy policy|Modern Slavery Act Transparency Statement|Sitemap|About US| Contact US: help@patsnap.com