An image super-resolution reconstruction method driven by semantic segmentation
A super-resolution reconstruction and semantic segmentation technology, applied in image analysis, image enhancement, image data processing, etc., can solve problems such as difficult to simulate complex real scenes, achieve simple framework, easy implementation, and improve semantic segmentation accuracy Effect
- Summary
- Abstract
- Description
- Claims
- Application Information
AI Technical Summary
Problems solved by technology
Method used
Image
Examples
Embodiment Construction
[0042] The embodiments of the present invention will be described in detail below, but the protection scope of the present invention is not limited to the examples.
[0043]Using VDSR as the super-resolution network and Deeplab-V2 as the semantic segmentation network, respectively perform 4 times and 8 times reconstruction, and the low-resolution image is obtained by down-sampling the high-resolution image. The specific steps are as follows:
[0044] (1) Independently train the super-resolution network VDSR and the semantic segmentation network Deeplab-V2. Train the super-resolution network with DIV2K and PASCALVOC 2012; train the semantic segmentation network with PASCAL VOC 2012;
[0045] (2) The super-resolution network and the semantic segmentation network of cascade independent training, initialize the parameter of the corresponding part in the cascade network with the parameter in the step (1);
[0046] (3) Driven by the semantic segmentation task, the super-resolution ...
PUM
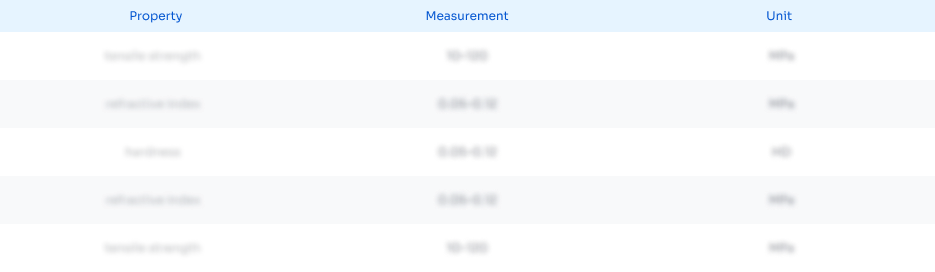
Abstract
Description
Claims
Application Information
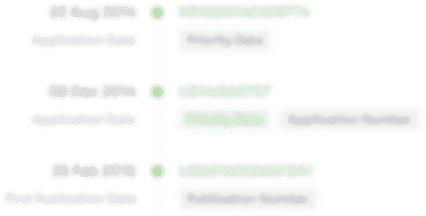
- R&D Engineer
- R&D Manager
- IP Professional
- Industry Leading Data Capabilities
- Powerful AI technology
- Patent DNA Extraction
Browse by: Latest US Patents, China's latest patents, Technical Efficacy Thesaurus, Application Domain, Technology Topic, Popular Technical Reports.
© 2024 PatSnap. All rights reserved.Legal|Privacy policy|Modern Slavery Act Transparency Statement|Sitemap|About US| Contact US: help@patsnap.com