An image segmentation method based on local energy functional and non-convex regular term combined with local entropy
A local energy and image segmentation technology, applied in the field of image processing, can solve problems such as poor grayscale image segmentation effect
- Summary
- Abstract
- Description
- Claims
- Application Information
AI Technical Summary
Problems solved by technology
Method used
Image
Examples
Embodiment 1
[0095] The present invention is used to segment three artificially synthesized images, such as figure 2 As shown in (a), there are three artificially synthesized images with uneven gray levels. The first and second images contain multiple gray levels, and the third image has uneven gray levels from top to bottom. The parameter settings are respectively : α=0.9, β=0.1, σ=3 μ=0.002*255 2 . from figure 2 It can be seen from (b) that the present invention can achieve effective segmentation for all three images with uneven gray levels.
Embodiment 2
[0097]In order to verify the effectiveness of the present invention, three kinds of images with uneven gray levels are used for comparative experiments, and the comparative experiments are respectively CV model and LBF model.
[0098] In this embodiment, three initial contours of different shapes are used to verify the sensitivity of the algorithm to the initial contour and the processing of heterogeneous regions. The parameters of the algorithm are selected as follows: α=0.1, β=1, and the control parameters of the length item are respectively selected as μ=0.001*255 2 , μ=0.003*255 2 and μ=0.01*255 2 , variance σ 1 = 3, σ 2 =5,σ 3 =2.
[0099] from image 3 and Figure 4 It can be seen that the superiority of the algorithm of the present invention can be seen through the final level set function graph and curve evolution results, wherein, Figure 4 columns of image 3 Corresponds to each column in . The invention can adapt to different initial contours of images, an...
Embodiment 3
[0101] In this embodiment, the present invention is compared with the CV model, the LBF model and the GLP model respectively, and the effects of the four methods on image segmentation are considered.
[0102] The specific parameters are set as follows: the first and second images α=0.25, β=0.75, μ=0.001*255 2 , σ=4, the third image α=0.1, β=0.9, μ=0.002*255 2 , σ=2.
[0103] Such as Figure 5 As shown, the first column is the image curve initialization position, the second column is the CV model segmentation result, the third column is the LBF model segmentation result, the fourth column is the GLP model segmentation result, and the fifth column is the segmentation result of the present invention. Table 1 shows the number of iterations and processing time of different algorithms in the comparative experiment, and Table 2 shows the comparison results of JS coefficients and DC coefficients of different algorithms.
[0104]
[0105]
[0106] Table 1
[0107]
[0108]...
PUM
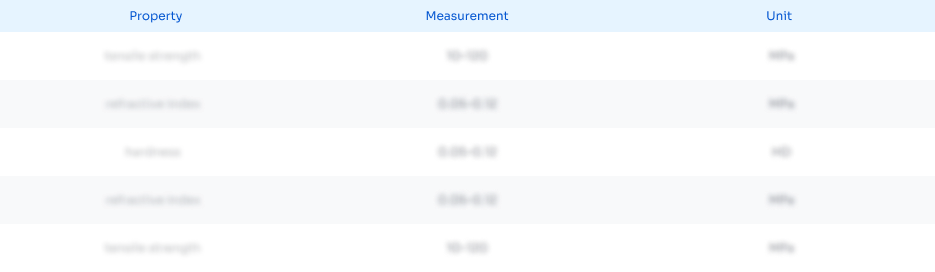
Abstract
Description
Claims
Application Information
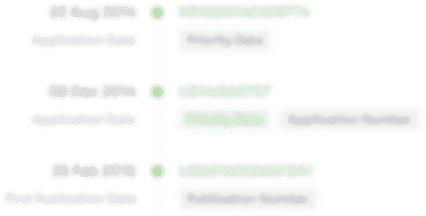
- R&D
- Intellectual Property
- Life Sciences
- Materials
- Tech Scout
- Unparalleled Data Quality
- Higher Quality Content
- 60% Fewer Hallucinations
Browse by: Latest US Patents, China's latest patents, Technical Efficacy Thesaurus, Application Domain, Technology Topic, Popular Technical Reports.
© 2025 PatSnap. All rights reserved.Legal|Privacy policy|Modern Slavery Act Transparency Statement|Sitemap|About US| Contact US: help@patsnap.com