An image semantic segmentation method based on an area and depth residual error network
A residual and network technology, applied in the field of computer vision, can solve problems such as rough segmentation boundaries and achieve good segmentation results
- Summary
- Abstract
- Description
- Claims
- Application Information
AI Technical Summary
Problems solved by technology
Method used
Image
Examples
Embodiment Construction
[0025] The present invention will be further described below in conjunction with the accompanying drawings and specific embodiments. A method for image semantic segmentation based on region and depth residual network, its specific implementation steps are as follows:
[0026] (S1): Extract candidate regions.
[0027] On the basis of Selective Search, use over-segmentation to divide the original image into multiple original regions, calculate the similarity between regions according to the color, texture, size and overlap of regions, and merge the most similar regions in turn, and repeat this process all the time Operate until merged into one area, so as to obtain candidate areas of different levels, and filter a certain number of candidate areas by setting the minimum size of the area. In the SIFT FLOW dataset and the PASCAL Context dataset, the minimum size set by the present invention is 100 pixels and 400 pixels respectively, and finally the average number of candidate reg...
PUM
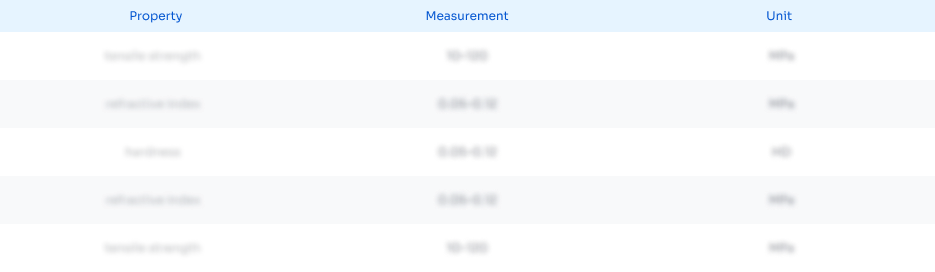
Abstract
Description
Claims
Application Information
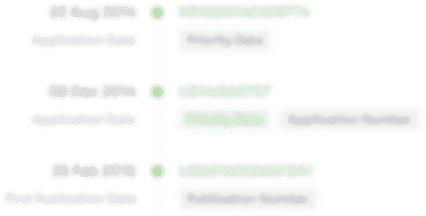
- R&D Engineer
- R&D Manager
- IP Professional
- Industry Leading Data Capabilities
- Powerful AI technology
- Patent DNA Extraction
Browse by: Latest US Patents, China's latest patents, Technical Efficacy Thesaurus, Application Domain, Technology Topic, Popular Technical Reports.
© 2024 PatSnap. All rights reserved.Legal|Privacy policy|Modern Slavery Act Transparency Statement|Sitemap|About US| Contact US: help@patsnap.com