Image semantic segmentation method based on local and global feature enhancement modules
A semantic segmentation and global feature technology, applied in image analysis, image enhancement, image coding, etc., can solve the problems of error-prone pixel classification and low segmentation accuracy of small-scale objects, so as to improve the segmentation effect, stabilize the segmentation performance, and achieve good segmentation. The effect of robustness
- Summary
- Abstract
- Description
- Claims
- Application Information
AI Technical Summary
Problems solved by technology
Method used
Image
Examples
Embodiment Construction
[0018] The present invention provides an image semantic segmentation method based on local and global feature enhancement modules, and the described embodiments are only part of the embodiments of the present invention. The flow process of the inventive method is as figure 1 , the image data is extracted through the encoder composed of the residual stage and the local feature enhancement module, and the final segmented image is generated by the decoder composed of the global feature enhancement module and the feature fusion operation, which is specifically divided into the following steps:
[0019] 1) Obtain the Cityscapes dataset, select and make the training set images, verification set image samples and label pictures required for the semantic segmentation task, for training and evaluation of the segmentation model.
[0020] 2) Perform data enhancement on the training set images, standardize the sample images in the training set images and verification set images respective...
PUM
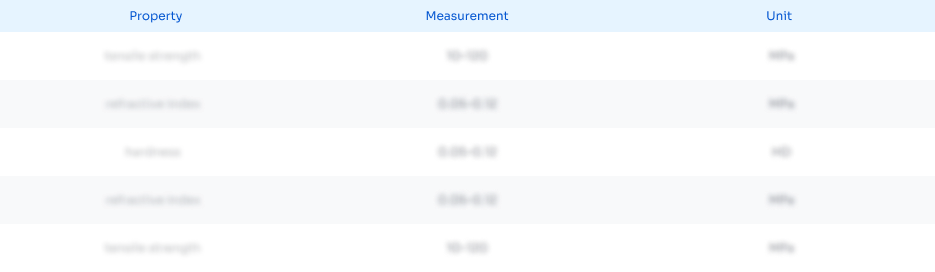
Abstract
Description
Claims
Application Information
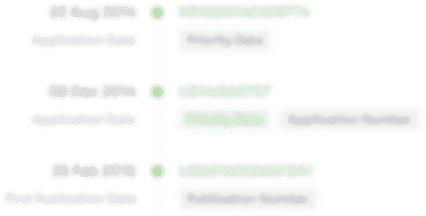
- Generate Ideas
- Intellectual Property
- Life Sciences
- Materials
- Tech Scout
- Unparalleled Data Quality
- Higher Quality Content
- 60% Fewer Hallucinations
Browse by: Latest US Patents, China's latest patents, Technical Efficacy Thesaurus, Application Domain, Technology Topic, Popular Technical Reports.
© 2025 PatSnap. All rights reserved.Legal|Privacy policy|Modern Slavery Act Transparency Statement|Sitemap|About US| Contact US: help@patsnap.com