Semantic image segmentation method based on multichannel convolutional neural network
A convolutional neural network and image segmentation technology, applied in the field of pattern recognition, can solve problems such as ignoring the shallow features of the network, achieve the effect of enriching global information, increasing the receptive field, and improving segmentation performance
- Summary
- Abstract
- Description
- Claims
- Application Information
AI Technical Summary
Problems solved by technology
Method used
Image
Examples
Embodiment Construction
[0028] The present invention will be further explained below in conjunction with the accompanying drawings.
[0029] A semantic image segmentation method based on a multi-channel convolutional neural network, comprising the steps of:
[0030] Step 1, construct a multi-channel convolutional neural network model, which contains 6 channels in total, and realize the fusion of shallow and deep features by "summing" the output of each channel. Compared with the single-channel network, this structure makes the final extracted features contain more information.
[0031] Such as figure 1 As shown, the multi-channel convolutional neural network model includes an input layer, a convolutional layer, a pooling layer, an activation function layer, a bilinear interpolation amplification layer, and a loss layer. The multi-channel convolutional neural network model has a total of 6 channels, which are A channel, B channel, C channel, D channel, E channel and F channel. The structure and para...
PUM
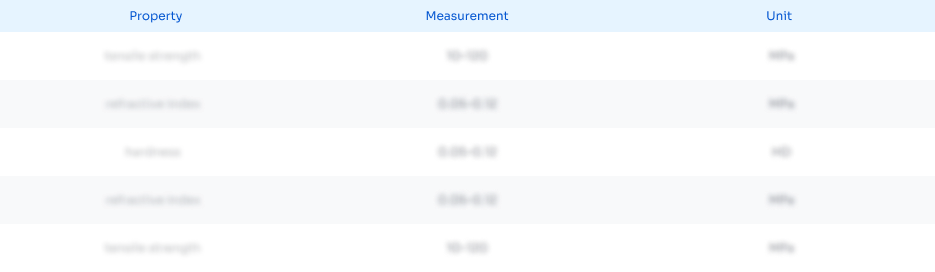
Abstract
Description
Claims
Application Information
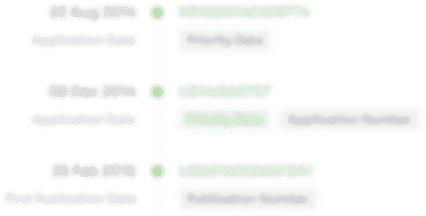
- R&D Engineer
- R&D Manager
- IP Professional
- Industry Leading Data Capabilities
- Powerful AI technology
- Patent DNA Extraction
Browse by: Latest US Patents, China's latest patents, Technical Efficacy Thesaurus, Application Domain, Technology Topic, Popular Technical Reports.
© 2024 PatSnap. All rights reserved.Legal|Privacy policy|Modern Slavery Act Transparency Statement|Sitemap|About US| Contact US: help@patsnap.com