Fabric surface defect detection method based on convolutional neural network
A convolutional neural network and defect detection technology, applied in the field of fabric surface defect detection, can solve the problems of time-consuming and labor-intensive execution process, and the accuracy and speed are difficult to meet the requirements, achieving high training efficiency, reducing classification difficulty, strong adaptability and robustness. awesome effect
- Summary
- Abstract
- Description
- Claims
- Application Information
AI Technical Summary
Problems solved by technology
Method used
Image
Examples
Embodiment 1
[0048] Embodiment 1: a kind of fabric surface defect detection method based on convolutional neural network of the present embodiment comprises the following steps:
[0049] S1. Collection and labeling of data sets: collect and mark images of several types of fabric surface defect sample sets, and collect one type of normal samples for labeling, and use the collected above sample images as a data set;
[0050] S2. GroundTruth for making defect sample images in the data set: divide all defect sample images in the data set into a training set and a verification set in a ratio of 8:2;
[0051] S3, building a convolutional neural network model;
[0052] S4, training the convolutional neural network model to obtain the optimal model;
[0053] S5. Collect the defect image of the fabric online, input the image of the fabric to be detected into the above-mentioned trained convolutional neural network model for image segmentation, and realize online automatic detection through the con...
PUM
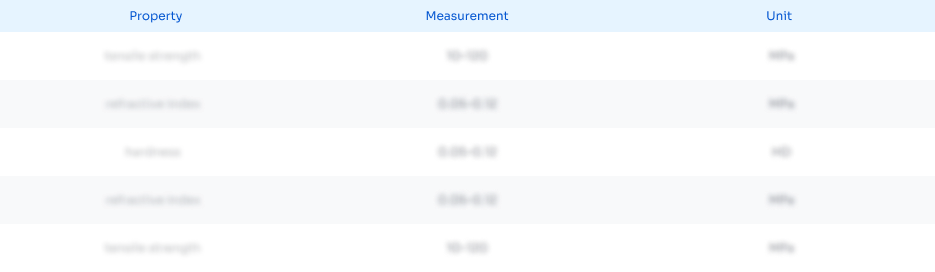
Abstract
Description
Claims
Application Information
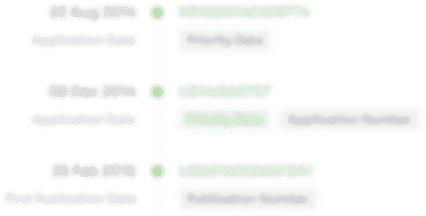
- R&D Engineer
- R&D Manager
- IP Professional
- Industry Leading Data Capabilities
- Powerful AI technology
- Patent DNA Extraction
Browse by: Latest US Patents, China's latest patents, Technical Efficacy Thesaurus, Application Domain, Technology Topic, Popular Technical Reports.
© 2024 PatSnap. All rights reserved.Legal|Privacy policy|Modern Slavery Act Transparency Statement|Sitemap|About US| Contact US: help@patsnap.com