Safety semi-supervised extreme learning machine classification method based on collaborative representation
An ELM and collaborative representation technology, applied in the field of pattern recognition, which can solve problems such as few, design, etc.
- Summary
- Abstract
- Description
- Claims
- Application Information
AI Technical Summary
Problems solved by technology
Method used
Image
Examples
Embodiment Construction
[0031] Describe in detail below in conjunction with accompanying drawing the classification method of the safety semi-supervised extreme learning machine of the present invention based on cooperative representation, figure 1 for the implementation flow chart.
[0032] Such as figure 1 , the implementation of the method of the present invention mainly includes four steps: (1) use the labeled sample to train the basic ELM classifier and the collaborative representation classifier; (2) utilize the reconstruction error of the unlabeled sample and the output consistency of the base classifier Construct the sample risk item; (3) combine the risk item matrix obtained in (2) into the loss function of the semi-supervised ELM; (4) perform a classification test on the semi-supervised ELM with a security mechanism, and draw conclusions compared to other methods.
[0033] Each step will be described in detail below one by one.
[0034] Step 1: Train the basic ELM classifier and collabor...
PUM
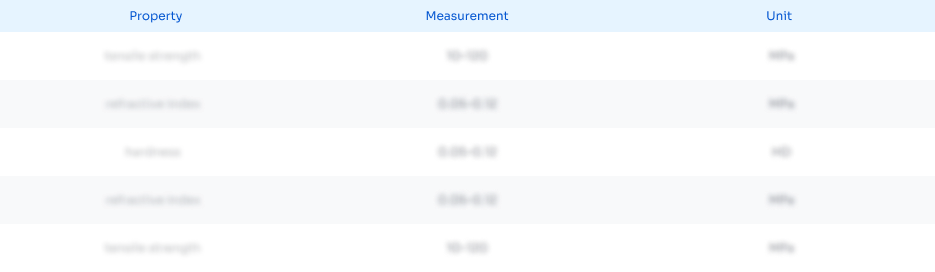
Abstract
Description
Claims
Application Information
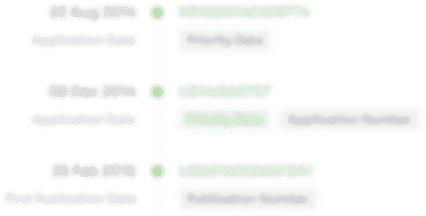
- R&D Engineer
- R&D Manager
- IP Professional
- Industry Leading Data Capabilities
- Powerful AI technology
- Patent DNA Extraction
Browse by: Latest US Patents, China's latest patents, Technical Efficacy Thesaurus, Application Domain, Technology Topic, Popular Technical Reports.
© 2024 PatSnap. All rights reserved.Legal|Privacy policy|Modern Slavery Act Transparency Statement|Sitemap|About US| Contact US: help@patsnap.com