Semi-supervised EFL classification method based on graph balance regularization
A technology of extreme learning machine and classification method, which is applied in the field of EEG signal classification, can solve the problems of not comprehensively considering the influence of training models, single consistent composition, etc., to achieve the effect of improving classification performance and solving time-consuming training
- Summary
- Abstract
- Description
- Claims
- Application Information
AI Technical Summary
Problems solved by technology
Method used
Image
Examples
Embodiment Construction
[0035] Describe in detail the semi-supervised extreme learning machine classification method based on graph balance regularization of the present invention below in conjunction with accompanying drawing, figure 1 for the implementation flow chart.
[0036] Such as figure 1 , the implementation of the method of the present invention mainly includes four steps: (1) train the basic ELM classifier with labeled samples, and predict the unlabeled training samples; The adjacency graph of label consistency; (3) Use the adjacency graph of label consistency and the original information similarity graph to form a new regular term and add it to the loss function for training; The learning machine conducts classification tests and draws conclusions compared to other methods.
[0037] Each step will be described in detail below one by one.
[0038] Step (1), use the 24-dimensional EEG signal of BCI to train the base classifier F of the extreme learning machine, and predict the unlabeled ...
PUM
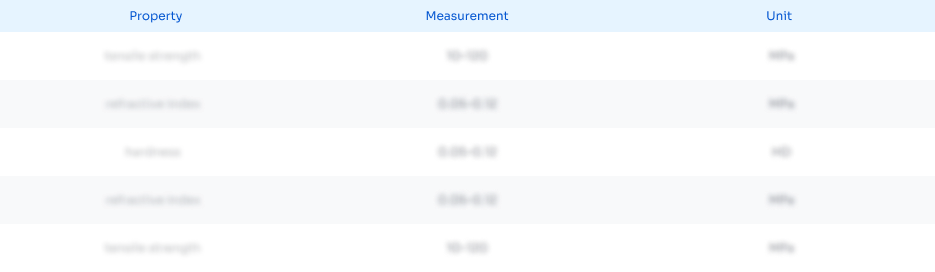
Abstract
Description
Claims
Application Information
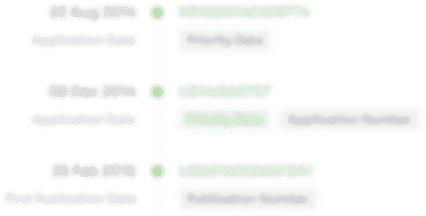
- R&D Engineer
- R&D Manager
- IP Professional
- Industry Leading Data Capabilities
- Powerful AI technology
- Patent DNA Extraction
Browse by: Latest US Patents, China's latest patents, Technical Efficacy Thesaurus, Application Domain, Technology Topic, Popular Technical Reports.
© 2024 PatSnap. All rights reserved.Legal|Privacy policy|Modern Slavery Act Transparency Statement|Sitemap|About US| Contact US: help@patsnap.com