traffic real-time classification method based on a shell vector type SVM incremental learning model
A technology of incremental learning and classification methods, applied in the field of incremental learning algorithms, can solve the problems of low incremental learning efficiency, long training time, poor real-time performance, etc., to ensure classification accuracy and timeliness, and reduce training time , to achieve the effect of incremental learning
- Summary
- Abstract
- Description
- Claims
- Application Information
AI Technical Summary
Problems solved by technology
Method used
Image
Examples
Embodiment Construction
[0052] Below in conjunction with accompanying drawing and embodiment the present invention will be described in further detail:
[0053] The characteristics of network traffic have obvious self-similarity, self-dependence and high dimensionality. Therefore, the present invention uses the FCBF algorithm based on symmetric uncertainty to delete redundant and irrelevant features of the high-dimensional training set, and then linearly superimposes the PCA algorithm to perform dimension reduction processing to obtain a new training set, and then trains the SVM model to realize the network Classification of traffic. When implementing incremental learning, the same data preprocessing is used for real-time network traffic, and then the union of new sample data and shell vectors that violate the KKT condition is used as a new training set to retrain the SVM model, thereby realizing incremental learning of SVM.
[0054] The invention is a real-time traffic classification method based o...
PUM
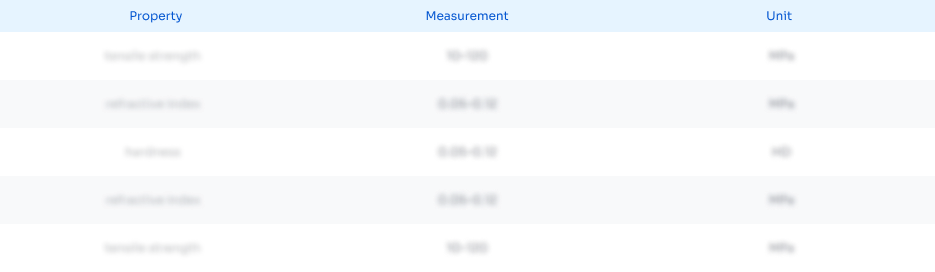
Abstract
Description
Claims
Application Information
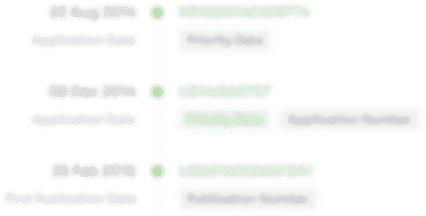
- R&D
- Intellectual Property
- Life Sciences
- Materials
- Tech Scout
- Unparalleled Data Quality
- Higher Quality Content
- 60% Fewer Hallucinations
Browse by: Latest US Patents, China's latest patents, Technical Efficacy Thesaurus, Application Domain, Technology Topic, Popular Technical Reports.
© 2025 PatSnap. All rights reserved.Legal|Privacy policy|Modern Slavery Act Transparency Statement|Sitemap|About US| Contact US: help@patsnap.com