A deep learning training sample optimization method
A training sample and deep learning technology, applied in the field of deep learning training sample optimization, can solve the problems of deep learning training sample generation efficiency and quality impact, unstable training sample training results, inaccurate and stable training results, etc., and shorten the training time. , eliminate the effect of occlusion, and eliminate the effect of boundary errors
- Summary
- Abstract
- Description
- Claims
- Application Information
AI Technical Summary
Problems solved by technology
Method used
Image
Examples
Embodiment Construction
[0021] The following will clearly and completely describe the technical solutions in the embodiments of the present invention with reference to the accompanying drawings in the embodiments of the present invention. Obviously, the described embodiments are only some, not all, embodiments of the present invention. Based on the embodiments of the present invention, all other embodiments obtained by persons of ordinary skill in the art without making creative efforts belong to the protection scope of the present invention.
[0022] according to figure 1 As shown, this embodiment proposes a deep learning training sample optimization method, which is characterized in that it includes the following steps:
[0023] Step 1: Obtain two sets of original images of the same deep learning training samples, and then use the findContours function to find the contours in the original images of the original images of one set of deep learning training samples, and use the findContours function t...
PUM
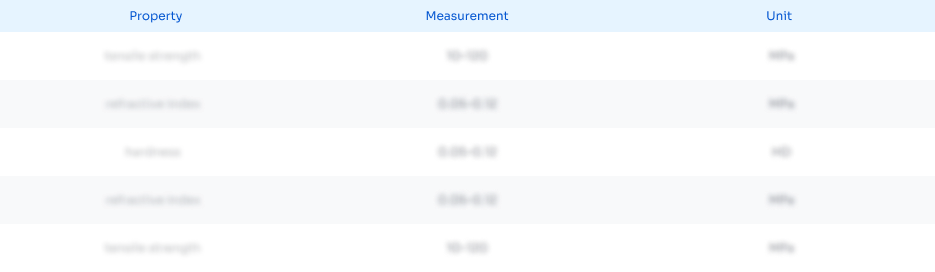
Abstract
Description
Claims
Application Information
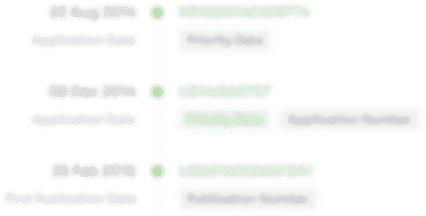
- R&D Engineer
- R&D Manager
- IP Professional
- Industry Leading Data Capabilities
- Powerful AI technology
- Patent DNA Extraction
Browse by: Latest US Patents, China's latest patents, Technical Efficacy Thesaurus, Application Domain, Technology Topic, Popular Technical Reports.
© 2024 PatSnap. All rights reserved.Legal|Privacy policy|Modern Slavery Act Transparency Statement|Sitemap|About US| Contact US: help@patsnap.com