Single-image super-resolution reconstruction algorithm based on multi-scale residual error learning network
A learning network, multi-scale technology, applied in the field of image processing, can solve the problems of increasing computational complexity and memory consumption
- Summary
- Abstract
- Description
- Claims
- Application Information
AI Technical Summary
Problems solved by technology
Method used
Image
Examples
specific Embodiment approach
[0012] A single image super-resolution reconstruction algorithm based on a multi-scale residual learning network, such as figure 1 As shown, it mainly consists of three parts: feature extraction block (FBlock), multi-scale information block (MFBlock) and reconstruction block (RBlock). Y and X represent the input and output of the MFN network, respectively.
[0013] A. Feature extraction block (FBlock)
[0014] A feature extraction block consisting of two layers of 3×3 convolutions extracts a feature map F from the original low-resolution image Y 0 , as shown in formula (1):
[0015] f 0 = f et (Y) (1)
[0016] In formula (1), f et Indicates the feature extraction function, F 0 Represents the feature channel extracted by two layers of convolution and sent to the first multi-scale information block, assuming that n multi-scale information blocks are stacked as the mapping process of residual information, this process is shown in formula (2):
[0017] f k =D k (F k-1 ),...
PUM
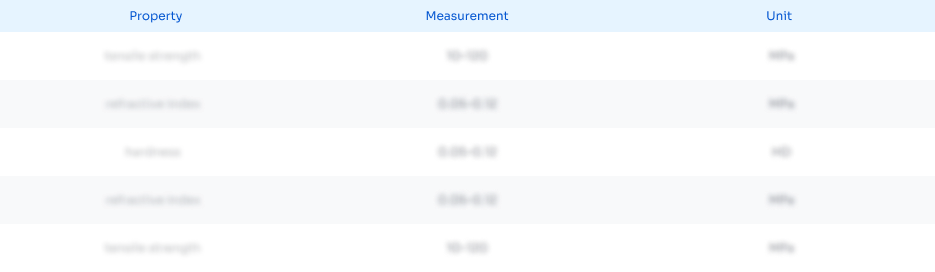
Abstract
Description
Claims
Application Information
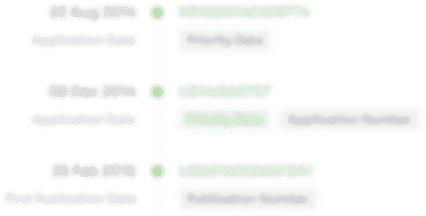
- R&D Engineer
- R&D Manager
- IP Professional
- Industry Leading Data Capabilities
- Powerful AI technology
- Patent DNA Extraction
Browse by: Latest US Patents, China's latest patents, Technical Efficacy Thesaurus, Application Domain, Technology Topic.
© 2024 PatSnap. All rights reserved.Legal|Privacy policy|Modern Slavery Act Transparency Statement|Sitemap