Semantic segmentation improved algorithm based on point cloud local structure
A local structure and semantic segmentation technology, applied in computing, image analysis, image data processing, etc., can solve the problem of not making full use of local neighborhoods and achieve good robustness
- Summary
- Abstract
- Description
- Claims
- Application Information
AI Technical Summary
Problems solved by technology
Method used
Image
Examples
Embodiment Construction
[0028] Below in conjunction with accompanying drawing and specific embodiment the present invention is described in further detail:
[0029] The present invention provides an improved semantic segmentation algorithm based on the local structure of the point cloud, which improves the accuracy rate to 80.6% under the S3DIS data set, and assists ORB-SLAM2 with the function of dense point cloud mapping, so that the SLAM system has the capability of semantic mapping Function.
[0030] The present invention provides an improved semantic segmentation algorithm based on the local structure of the point cloud, and the specific implementation steps are as follows;
[0031] Step 1: Learning Local Geometry
[0032] At the front end of the KCNet network, we take inspiration from kernel-correlation-based point cloud registration, and consider the local neighborhood of points as a source, and a set of learnable points (i.e., kernels) as a reference to characterize certain types of local ge...
PUM
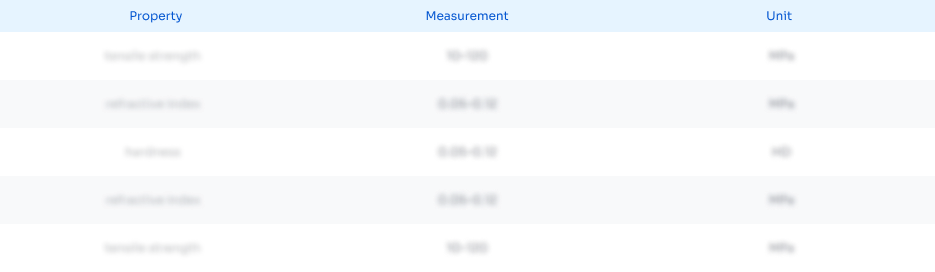
Abstract
Description
Claims
Application Information
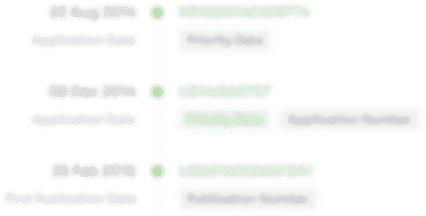
- R&D Engineer
- R&D Manager
- IP Professional
- Industry Leading Data Capabilities
- Powerful AI technology
- Patent DNA Extraction
Browse by: Latest US Patents, China's latest patents, Technical Efficacy Thesaurus, Application Domain, Technology Topic, Popular Technical Reports.
© 2024 PatSnap. All rights reserved.Legal|Privacy policy|Modern Slavery Act Transparency Statement|Sitemap|About US| Contact US: help@patsnap.com