Visual odometer method based on end-to-end semi-supervised generative adversarial network
A visual odometry and semi-supervised technology, applied in the field of visual odometry calculation method, can solve the problems of limited usage scenarios, limited precision, and difficulty in obtaining data marked with geometric information, so as to avoid local features and improve matching accuracy.
- Summary
- Abstract
- Description
- Claims
- Application Information
AI Technical Summary
Problems solved by technology
Method used
Image
Examples
Embodiment Construction
[0032] The following embodiments will further illustrate the present invention in conjunction with the accompanying drawings.
[0033] Embodiments of the present invention include the following steps:
[0034] 1) Construct a feature generation network. The specific method is: in the deep feature point detection and descriptor extraction method, the feature point and the descriptor are considered separately, and the feature point and the depth descriptor are generated simultaneously by using the generation network, and the speed can be optimized. Based on the SIFT operator, the feature generation network is divided into two functions: the feature point detector and the depth feature descriptor extraction. The RGB image is used as input, and the pixel-level feature point probability map and depth feature descriptor are generated through the encoder and decoder; When the feature point detector is designed, calculation efficiency and real-time performance are considered, so the ne...
PUM
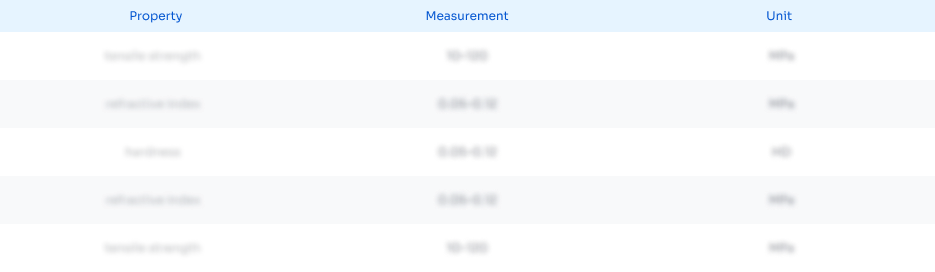
Abstract
Description
Claims
Application Information
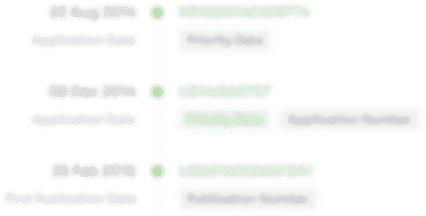
- R&D
- Intellectual Property
- Life Sciences
- Materials
- Tech Scout
- Unparalleled Data Quality
- Higher Quality Content
- 60% Fewer Hallucinations
Browse by: Latest US Patents, China's latest patents, Technical Efficacy Thesaurus, Application Domain, Technology Topic, Popular Technical Reports.
© 2025 PatSnap. All rights reserved.Legal|Privacy policy|Modern Slavery Act Transparency Statement|Sitemap|About US| Contact US: help@patsnap.com