Model training method, text classification method and related devices
A technology for model training and training samples, applied in the field of machine learning, can solve problems such as poor model training effect, weakly supervised learning training samples, and cost of large manpower and material resources, so as to reduce learning label errors and inaccurate sample information, Improve the effect of training
- Summary
- Abstract
- Description
- Claims
- Application Information
AI Technical Summary
Problems solved by technology
Method used
Image
Examples
Embodiment Construction
[0041] In order to make the purpose, technical solutions and advantages of the embodiments of this application clearer, the technical solutions in the embodiments of this application will be described clearly and completely in conjunction with the drawings in the embodiments of this application. Obviously, the described embodiments It is a part of the embodiments of this application, but not all the embodiments. The components of the embodiments of the present application generally described and shown in the drawings herein may be arranged and designed in various different configurations.
[0042] Therefore, the following detailed description of the embodiments of the present application provided in the accompanying drawings is not intended to limit the scope of the claimed application, but merely represents selected embodiments of the present application. Based on the embodiments in this application, all other embodiments obtained by those of ordinary skill in the art without cr...
PUM
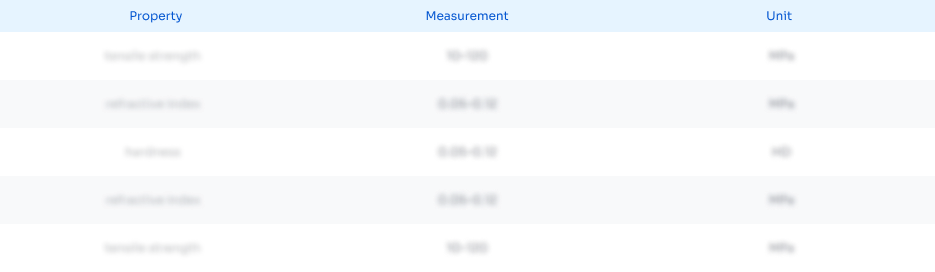
Abstract
Description
Claims
Application Information
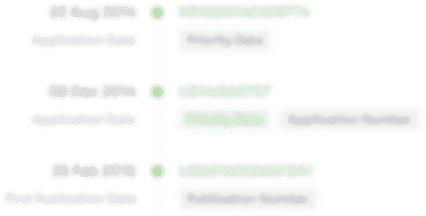
- Generate Ideas
- Intellectual Property
- Life Sciences
- Materials
- Tech Scout
- Unparalleled Data Quality
- Higher Quality Content
- 60% Fewer Hallucinations
Browse by: Latest US Patents, China's latest patents, Technical Efficacy Thesaurus, Application Domain, Technology Topic, Popular Technical Reports.
© 2025 PatSnap. All rights reserved.Legal|Privacy policy|Modern Slavery Act Transparency Statement|Sitemap|About US| Contact US: help@patsnap.com