Image denoising method based on generative adversarial network
An image and network technology, applied in the fields of image analysis, deep learning and computer vision, which can solve the problems of blurred edges, unknown features, and loss.
- Summary
- Abstract
- Description
- Claims
- Application Information
AI Technical Summary
Problems solved by technology
Method used
Image
Examples
Embodiment Construction
[0055] The present invention will be described in detail below in conjunction with the accompanying drawings and specific embodiments.
[0056] The present invention provides an image denoising method based on generation confrontation network, such as figure 2 As shown, the generator learns an end-to-end mapping from noisy images to real images through multiple layers of convolution and sub-pixel layers, and the discriminator supervises and corrects the training of the generator. The generator uses residual learning to deepen the number of network layers, prevent network degradation, and increase the number of learned features. The joint loss function reduces the checkerboard effect in the image denoising process. When the denoised image is far from the noise-free image A large loss value will be generated, and the discriminator supervises the network to train in a better direction through this value, so that the denoised image generated by the generator is more in line with ...
PUM
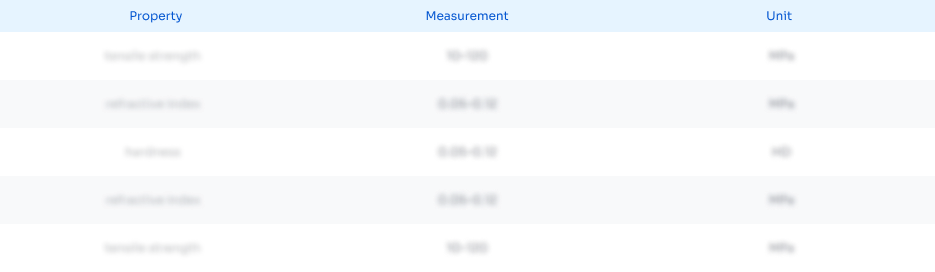
Abstract
Description
Claims
Application Information
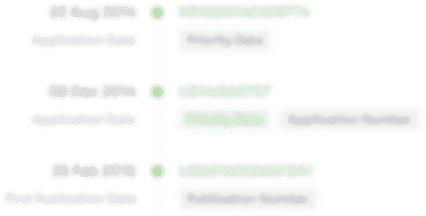
- R&D Engineer
- R&D Manager
- IP Professional
- Industry Leading Data Capabilities
- Powerful AI technology
- Patent DNA Extraction
Browse by: Latest US Patents, China's latest patents, Technical Efficacy Thesaurus, Application Domain, Technology Topic, Popular Technical Reports.
© 2024 PatSnap. All rights reserved.Legal|Privacy policy|Modern Slavery Act Transparency Statement|Sitemap|About US| Contact US: help@patsnap.com