Image deblurring method based on generative adversarial network
A deblurring and network technology, applied in the field of image processing, can solve the problems of slow running speed and inability to use the blur function, and achieve the effect of good deblurring effect, reducing checkerboard effect and overcoming checkerboard effect.
- Summary
- Abstract
- Description
- Claims
- Application Information
AI Technical Summary
Problems solved by technology
Method used
Image
Examples
Embodiment Construction
[0025] Specific implementation plan
[0026] The following describes the embodiments of the present invention in detail with reference to the accompanying drawings:
[0027] Reference figure 1 , The implementation steps of this example are as follows:
[0028] Step 1. Select the experimental data set and determine the training data set and test data set related to it.
[0029] The training set in the GOPRO dataset is selected as the source of training data, and the data in the training set is expanded by flipping, horizontal and vertical rotation in turn, and the original size of the training dataset is 1280*720 from the expanded training dataset. The image is randomly cropped into an image with a size of 256*256 as the final training data.
[0030] The test set in the GOPRO data set is selected as the test data.
[0031] Step 2. Build a generation network.
[0032] Reference figure 2 , The generation network built for this example includes a 15-layer structure of two convolutional lay...
PUM
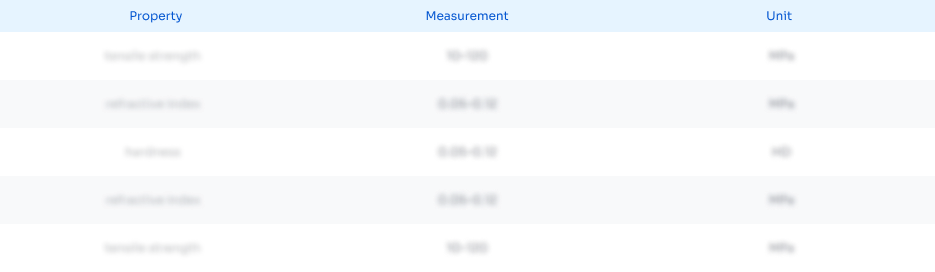
Abstract
Description
Claims
Application Information
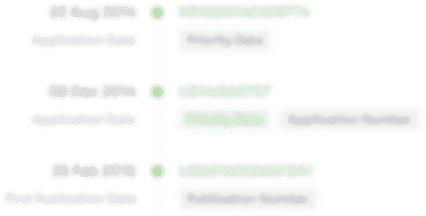
- R&D Engineer
- R&D Manager
- IP Professional
- Industry Leading Data Capabilities
- Powerful AI technology
- Patent DNA Extraction
Browse by: Latest US Patents, China's latest patents, Technical Efficacy Thesaurus, Application Domain, Technology Topic, Popular Technical Reports.
© 2024 PatSnap. All rights reserved.Legal|Privacy policy|Modern Slavery Act Transparency Statement|Sitemap|About US| Contact US: help@patsnap.com