HRTF Personalization Method Based on Sparse Principal Component Analysis
A sparse principal component and principal component analysis technology, applied in the field of HRTF personalization, can solve problems such as weak local correlation, no persuasiveness, and no consideration of the correlation of physiological parameters
- Summary
- Abstract
- Description
- Claims
- Application Information
AI Technical Summary
Problems solved by technology
Method used
Image
Examples
Embodiment Construction
[0038] refer to figure 1 . The HRTF personalization process for HRTF with azimuth angle θ=0° and elevation angle φ=0° is introduced in detail.
[0039] Step 1: Select HRTF of all subjects in the orientation (0°, 0°), conduct principal component analysis on the HRTF of this orientation, and obtain the principal component of this orientation:
[0040] (1) Select all subjects in the HRTF at the orientation (0°,0°) to form the vector H ij , where i is the subject sequence, j is the frequency number, and the number of subjects is m, where the azimuth angle θ=0°, the elevation angle φ=0°;
[0041] (2) to H ij Standardize as follows:
[0042]
[0043] in, for H ij Normalized head-related transfer function;
[0044] (3) Perform principal component analysis on the standardized head-related transfer function:
[0045]
[0046] Among them, P m×n is the m×n score matrix of principal component analysis; W is the n×n load matrix of principal component analysis, and T repre...
PUM
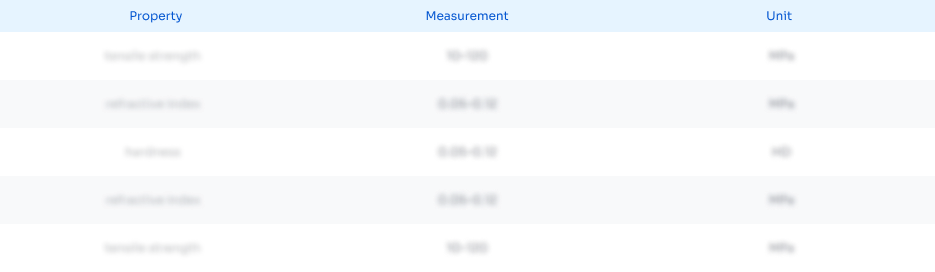
Abstract
Description
Claims
Application Information
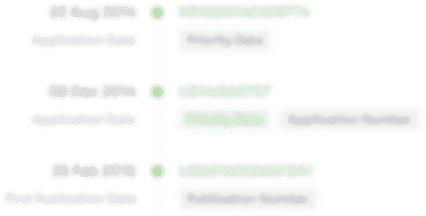
- R&D Engineer
- R&D Manager
- IP Professional
- Industry Leading Data Capabilities
- Powerful AI technology
- Patent DNA Extraction
Browse by: Latest US Patents, China's latest patents, Technical Efficacy Thesaurus, Application Domain, Technology Topic, Popular Technical Reports.
© 2024 PatSnap. All rights reserved.Legal|Privacy policy|Modern Slavery Act Transparency Statement|Sitemap|About US| Contact US: help@patsnap.com