A task assignment method based on deep learning inference for resource-constrained robots
A deep learning and task allocation technology, applied in the direction of resource allocation, instruments, electrical and digital data processing, etc., can solve the problem that the unknown characteristics of multi-robot system resources are not considered, the robot cannot be clearly known, and more work cannot be allocated to those with stronger abilities. It can reduce the data transmission delay, reduce the data transmission overhead, and optimize the execution time.
- Summary
- Abstract
- Description
- Claims
- Application Information
AI Technical Summary
Problems solved by technology
Method used
Image
Examples
Embodiment Construction
[0090] image 3 It is an overall flow chart of the present invention. Such as image 3 Shown, the present invention comprises the following steps:
[0091] The first step is to build a task distribution system composed of multiple robot nodes. Such as figure 1 As shown, the task distribution system is composed of various heterogeneous robot nodes (such as drones, ground robots, humanoid robots, etc.), and these heterogeneous robot nodes are interconnected through WIFI.
[0092] Robot nodes are divided into task robot nodes and collaborative robot nodes according to their functions. The task robot node refers to the initiator of the robot engineering task based on deep learning. The task robot node interacts with complex and harsh environments such as earthquake relief, mountainous areas, and floods to collect environmental data; the collaborative robot node refers to the rest of the robots in the robot environment. Responsible for supporting task robots and cooperating w...
PUM
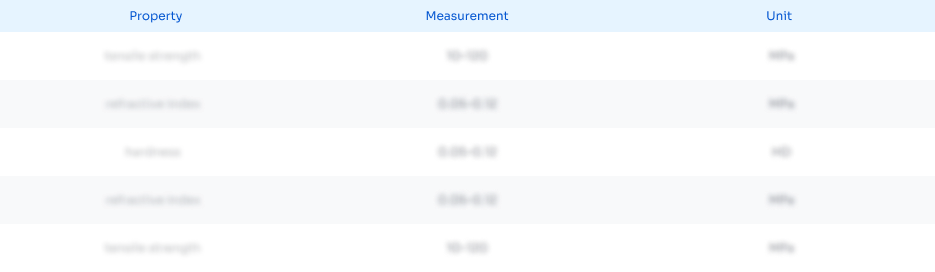
Abstract
Description
Claims
Application Information
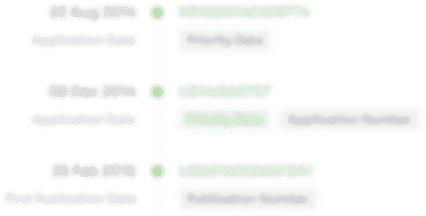
- R&D
- Intellectual Property
- Life Sciences
- Materials
- Tech Scout
- Unparalleled Data Quality
- Higher Quality Content
- 60% Fewer Hallucinations
Browse by: Latest US Patents, China's latest patents, Technical Efficacy Thesaurus, Application Domain, Technology Topic, Popular Technical Reports.
© 2025 PatSnap. All rights reserved.Legal|Privacy policy|Modern Slavery Act Transparency Statement|Sitemap|About US| Contact US: help@patsnap.com