Feature and instance joint transfer learning method in semi-supervised scene
A transfer learning, semi-supervised technique used in kernel methods, etc.
- Summary
- Abstract
- Description
- Claims
- Application Information
AI Technical Summary
Problems solved by technology
Method used
Image
Examples
Embodiment Construction
[0054] The present invention will be further described in detail below in conjunction with the embodiments and the accompanying drawings, but the embodiments of the present invention are not limited thereto.
[0055] The basic process of the present invention is as figure 1 As shown, the embodiment of the present invention provides a feature and instance joint transfer learning method in a semi-supervised scene, including the following steps:
[0056] Step 1: Define the data in the model as follows:
[0057] Given a field, it is known that the category of each data in this field is represented by "0" or "1", which is recorded as the source domain D s . The n samples included are expressed in the form of feature matrix and category label vector as Abbreviated as {x s ,y s}. Given another domain, denoted as the target domain D t . Among them, m samples form a labeled data set, which is denoted as The unlabeled dataset is denoted as where q=1,...,k. The two types of...
PUM
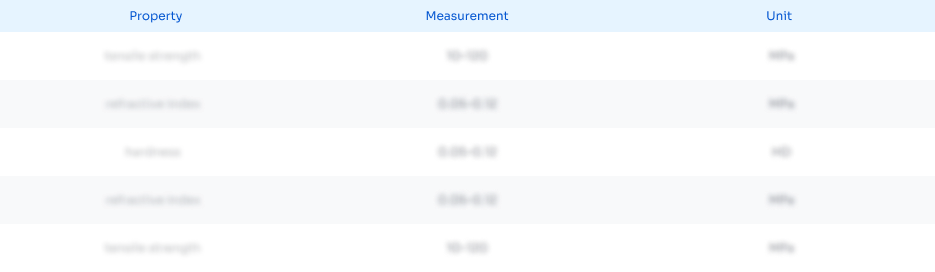
Abstract
Description
Claims
Application Information
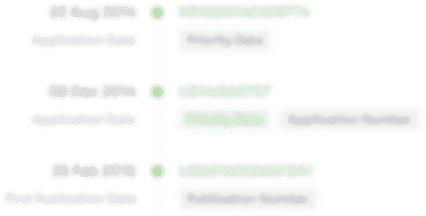
- R&D Engineer
- R&D Manager
- IP Professional
- Industry Leading Data Capabilities
- Powerful AI technology
- Patent DNA Extraction
Browse by: Latest US Patents, China's latest patents, Technical Efficacy Thesaurus, Application Domain, Technology Topic, Popular Technical Reports.
© 2024 PatSnap. All rights reserved.Legal|Privacy policy|Modern Slavery Act Transparency Statement|Sitemap|About US| Contact US: help@patsnap.com