Improved Scale Affine Projection Filtering Method Based on Generalized Correlation Induced Metric
A technique of generalized correlation and affine projection, applied in the field of sparse adaptive filtering, which can solve problems such as slow convergence of applications
- Summary
- Abstract
- Description
- Claims
- Application Information
AI Technical Summary
Problems solved by technology
Method used
Image
Examples
Embodiment Construction
[0041] The technical solution of the present invention will be further described in detail below in conjunction with the accompanying drawings, but the protection scope of the present invention is not limited to the following description.
[0042] Such as figure 1 As shown, the improved proportional affine projection filtering method based on the generalized correlation induction metric includes the following steps:
[0043] S1. The expected weight of the filter The transpose of the input signal u(n)=[u(n),u(n-1),...,u(n-M+1)] of the filter at instant n T ∈R M×1 Multiply and add the noise signal v(n) to get the desired output signal d(n):
[0044] d(n)=w 0 T u(n)+v(n);
[0045] In the formula, M represents the channel length;
[0046] S2. At each moment between instant n and instant n-K+1, repeat step S1 to obtain corresponding expected output signals d(n), d(n-1),...,d(n -K+1); and these desired output signals are formed into the desired output vector, and obtained: ...
PUM
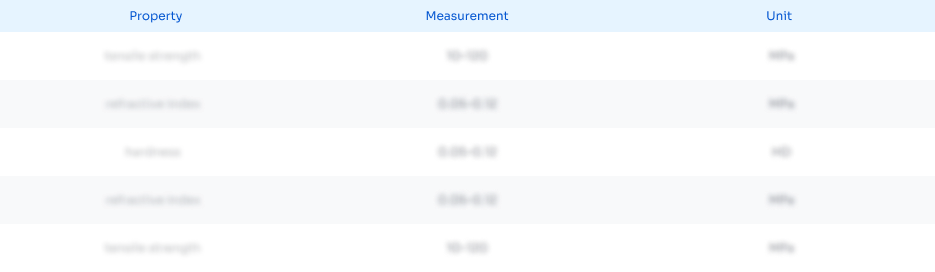
Abstract
Description
Claims
Application Information
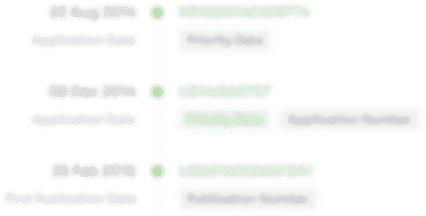
- R&D Engineer
- R&D Manager
- IP Professional
- Industry Leading Data Capabilities
- Powerful AI technology
- Patent DNA Extraction
Browse by: Latest US Patents, China's latest patents, Technical Efficacy Thesaurus, Application Domain, Technology Topic, Popular Technical Reports.
© 2024 PatSnap. All rights reserved.Legal|Privacy policy|Modern Slavery Act Transparency Statement|Sitemap|About US| Contact US: help@patsnap.com