Multi-modal robust feature learning model based on non-negative matrix factorization
A non-negative matrix decomposition and feature learning technology, applied in the computer field, can solve problems such as the adverse effects of multi-modal fusion features, and achieve the effect of excellent data representation performance
- Summary
- Abstract
- Description
- Claims
- Application Information
AI Technical Summary
Problems solved by technology
Method used
Image
Examples
Embodiment Construction
[0021] The technical solutions in the embodiments of the present invention will be clearly and completely described below in conjunction with the accompanying drawings in the embodiments of the present invention. Obviously, the described examples are only a part of the examples of the present invention, not all examples. Based on the examples in the present invention, all other examples obtained by those of ordinary skill in the art without creative work shall fall within the protection scope of the present invention.
[0022] The multi-modal robust feature learning model based on non-negative matrix factorization in the example of the present invention specifically includes the following steps:
[0023] The first step is to perform normalization and special value preprocessing on the data items of the multimodal data set
[0024] In the initial stage of the model, the multi-modal data set is preprocessed, and all the data attribute values are set to a linearly transformed non-nega...
PUM
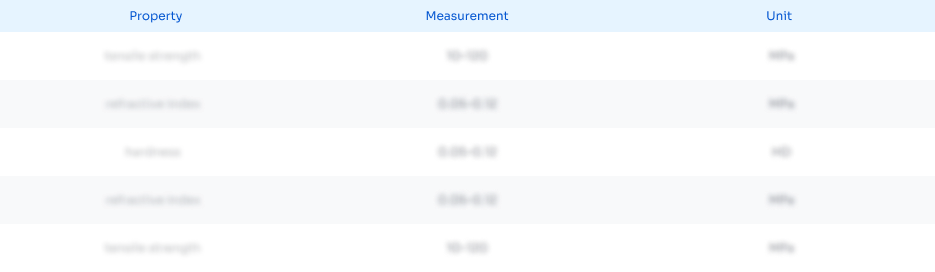
Abstract
Description
Claims
Application Information
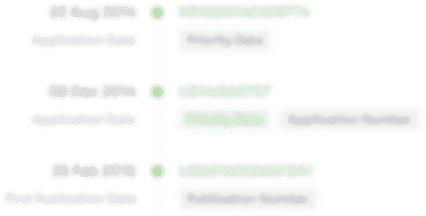
- R&D
- Intellectual Property
- Life Sciences
- Materials
- Tech Scout
- Unparalleled Data Quality
- Higher Quality Content
- 60% Fewer Hallucinations
Browse by: Latest US Patents, China's latest patents, Technical Efficacy Thesaurus, Application Domain, Technology Topic, Popular Technical Reports.
© 2025 PatSnap. All rights reserved.Legal|Privacy policy|Modern Slavery Act Transparency Statement|Sitemap|About US| Contact US: help@patsnap.com