An Unsupervised Anomaly Detection Method for Time Series Based on Conditional Regularized Flow Model
A technology of time series and anomaly detection, applied in neural learning methods, biological neural network models, digital data information retrieval, etc., can solve problems such as high false alarm rate, prone to false negatives, and complex distribution
- Summary
- Abstract
- Description
- Claims
- Application Information
AI Technical Summary
Problems solved by technology
Method used
Image
Examples
Embodiment Construction
[0020] In order to make the object, technical solution and advantages of the present invention clearer, the present invention will be further described in detail below in conjunction with the accompanying drawings and embodiments. It should be understood that the specific embodiments described here are only used to explain the present invention, and do not limit the protection scope of the present invention.
[0021] figure 1 An overall flow of a time series unsupervised anomaly detection method based on a conditional normalized flow model provided for the embodiment; figure 2 An overall framework of an unsupervised anomaly detection method for time series based on a conditional normalized flow model is provided for the embodiment.
[0022] see figure 1 and figure 2 , an unsupervised time series anomaly detection method based on a conditional normalized flow model provided by the embodiment, using a normalized flow model conditional on a historical observation sequence to...
PUM
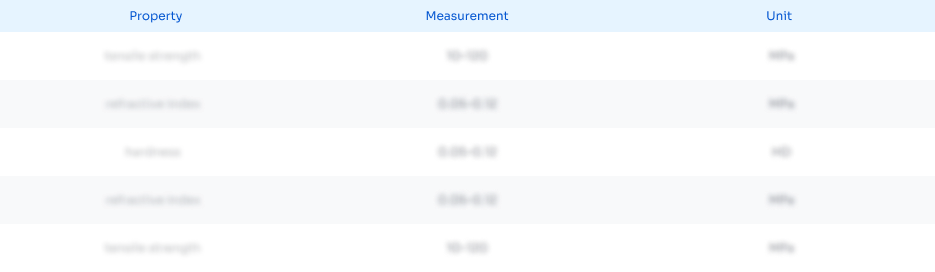
Abstract
Description
Claims
Application Information
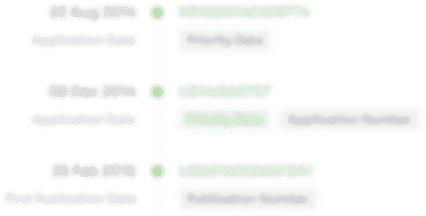
- R&D Engineer
- R&D Manager
- IP Professional
- Industry Leading Data Capabilities
- Powerful AI technology
- Patent DNA Extraction
Browse by: Latest US Patents, China's latest patents, Technical Efficacy Thesaurus, Application Domain, Technology Topic, Popular Technical Reports.
© 2024 PatSnap. All rights reserved.Legal|Privacy policy|Modern Slavery Act Transparency Statement|Sitemap|About US| Contact US: help@patsnap.com