Entity relationship classification method of unstructured text based on WordNet and IDF
An entity-relationship, unstructured technology, applied in unstructured text data retrieval, text database clustering/classification, neural learning methods, etc., can solve problems such as training data noise, data labeling errors, etc.
- Summary
- Abstract
- Description
- Claims
- Application Information
AI Technical Summary
Problems solved by technology
Method used
Image
Examples
Embodiment Construction
[0064] In order to make the object, technical solution and advantages of the present invention clearer, the present invention will be described in further detail below in conjunction with specific embodiments and with reference to the accompanying drawings.
[0065] The present invention proposes an entity relationship classification method for unstructured text based on WordNet and IDF, uses external information and internal information vectors to carry out semantic expansion on entities and sentences, and then uses segmental convolutional neural networks to extract fixed-length semantic features Vector, used to train the classifier, and finally can classify the entity relationship of unstructured text. The basic features of the present invention mainly include the following aspects: one is to add the external information of the entity, internal information and sentence structure information in the vector after the sentence is vectorized; the other is to use the convolutional ...
PUM
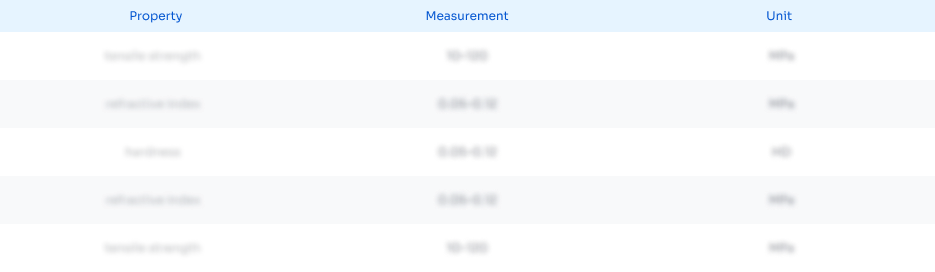
Abstract
Description
Claims
Application Information
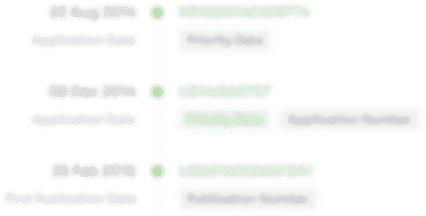
- R&D Engineer
- R&D Manager
- IP Professional
- Industry Leading Data Capabilities
- Powerful AI technology
- Patent DNA Extraction
Browse by: Latest US Patents, China's latest patents, Technical Efficacy Thesaurus, Application Domain, Technology Topic, Popular Technical Reports.
© 2024 PatSnap. All rights reserved.Legal|Privacy policy|Modern Slavery Act Transparency Statement|Sitemap|About US| Contact US: help@patsnap.com