Spatial pyramid graph convolutional network implementation method
A technology of spatial pyramid and convolutional network, applied in the field of spatial pyramid graph convolutional network implementation, can solve problems such as large amount of calculation, and achieve the effects of increasing expression ability, reducing computational complexity, and achieving remarkable performance.
- Summary
- Abstract
- Description
- Claims
- Application Information
AI Technical Summary
Problems solved by technology
Method used
Image
Examples
Embodiment Construction
[0039] Below in conjunction with accompanying drawing, further describe the present invention through embodiment, but do not limit the scope of the present invention in any way.
[0040] According to the characteristics of the full convolutional network, the present invention designs the generation method of the Laplacian matrix, and by decomposing it, the amount of calculation is significantly reduced; this change allows it to run directly on the original feature space, avoiding the mapping -Information loss caused by reverse mapping process. The present invention is a kind of spatial pyramid graph convolutional network, including such as figure 1 The graph convolution module shown is implemented by the following steps:
[0041] (1) Input the feature map X∈R extracted by the backbone network H×W×C ; where H, W, and C refer to the height, width, and number of channels of the feature map X, respectively, then H×W is the number of nodes in the feature map X, and C is the featu...
PUM
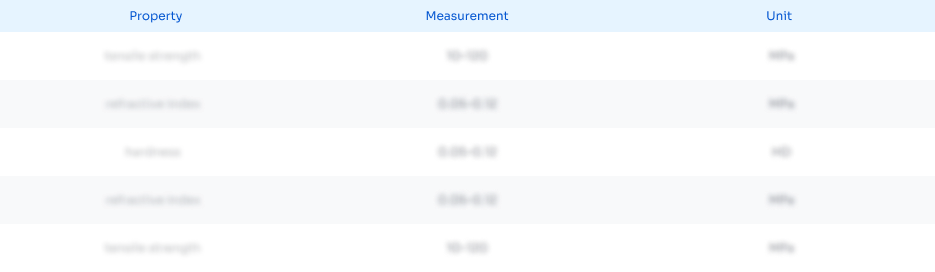
Abstract
Description
Claims
Application Information
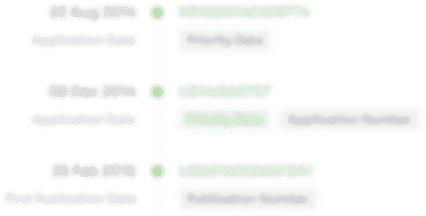
- R&D
- Intellectual Property
- Life Sciences
- Materials
- Tech Scout
- Unparalleled Data Quality
- Higher Quality Content
- 60% Fewer Hallucinations
Browse by: Latest US Patents, China's latest patents, Technical Efficacy Thesaurus, Application Domain, Technology Topic, Popular Technical Reports.
© 2025 PatSnap. All rights reserved.Legal|Privacy policy|Modern Slavery Act Transparency Statement|Sitemap|About US| Contact US: help@patsnap.com