Rainfall runoff simulation method for physical mechanism guided deep learning
A technology of deep learning and simulation methods, applied in machine learning, computational models, computer components, etc., can solve problems such as not fully understanding the causal relationship of scientific problems, low probability of extreme rainfall, and large flood peak flow simulation errors, etc. achieve the effect of avoiding non-monotonicity
- Summary
- Abstract
- Description
- Claims
- Application Information
AI Technical Summary
Problems solved by technology
Method used
Image
Examples
Embodiment Construction
[0041] In order to better understand the present invention, the present invention will be further described below in conjunction with the accompanying drawings and embodiments.
[0042] Such as figure 1 As shown, the present invention provides a physical mechanism-guided deep learning rainfall runoff simulation method. Technical scheme of the present invention specifically comprises the following steps:
[0043] Step 1, select a deep learning model for predicting simulated hydrological time series, such as a long short-term memory neural network (Long short-term memory, LSTM) model;
[0044] Step 2, according to the historical weather (precipitation p, maximum and minimum temperature T max ,T min , potential evapotranspiration e, solar radiation S, atmospheric pressure V p etc.), hydrology (runoff q), and time data, which are normalized and sorted as model input;
[0045]
[0046] In the formula, is the runoff value simulated by the deep learning method; f is the simul...
PUM
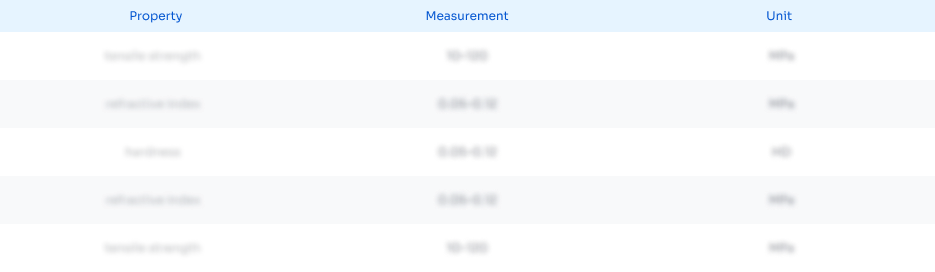
Abstract
Description
Claims
Application Information
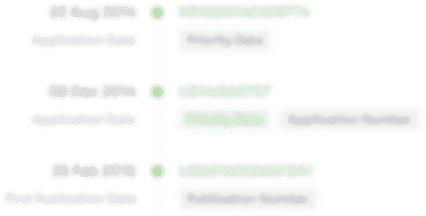
- R&D Engineer
- R&D Manager
- IP Professional
- Industry Leading Data Capabilities
- Powerful AI technology
- Patent DNA Extraction
Browse by: Latest US Patents, China's latest patents, Technical Efficacy Thesaurus, Application Domain, Technology Topic, Popular Technical Reports.
© 2024 PatSnap. All rights reserved.Legal|Privacy policy|Modern Slavery Act Transparency Statement|Sitemap|About US| Contact US: help@patsnap.com