Vehicle detection method based on improved YOLOv3
A vehicle detection and vehicle technology, which is applied in the field of computer vision, can solve the problems of insufficient real-time performance and precision, and detection time reduction, and achieve the effect of enriching the number, improving efficiency, and ensuring accuracy
- Summary
- Abstract
- Description
- Claims
- Application Information
AI Technical Summary
Problems solved by technology
Method used
Image
Examples
Embodiment Construction
[0060] Below in conjunction with accompanying drawing and specific embodiment, technical scheme of the present invention is described in detail:
[0061] The present invention aims to build a fast and efficient vehicle detection method, and the following are the specific implementation steps involved in the specific implementation of the present invention:
[0062] S1. Collect the original video and perform frame extraction and preprocessing to obtain an image set;
[0063] The target category to be learned in this example involves three categories: passenger car, SUV, and sedan.
[0064] Obtain real-time monitoring video of road vehicles as raw video data. The video recording involves four weathers: sunny, rainy, cloudy, and light fog; the video recording time is 8:00-18:00;
[0065] Frame images are extracted from raw video data in a timed manner as a raw image set. Get 6000 vehicle images under different weather and different time.
[0066] Noise reduction is performed ...
PUM
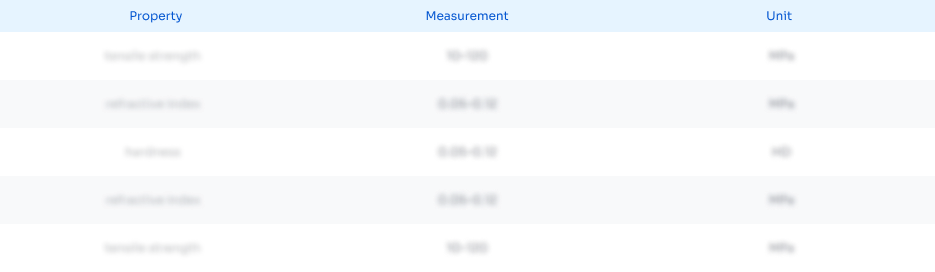
Abstract
Description
Claims
Application Information
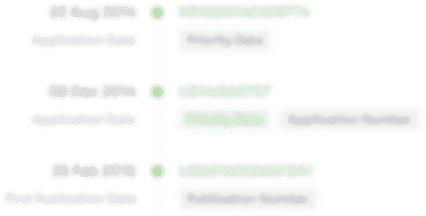
- R&D Engineer
- R&D Manager
- IP Professional
- Industry Leading Data Capabilities
- Powerful AI technology
- Patent DNA Extraction
Browse by: Latest US Patents, China's latest patents, Technical Efficacy Thesaurus, Application Domain, Technology Topic, Popular Technical Reports.
© 2024 PatSnap. All rights reserved.Legal|Privacy policy|Modern Slavery Act Transparency Statement|Sitemap|About US| Contact US: help@patsnap.com