Dense crowd counting algorithm based on cascaded high-resolution convolutional neural network
A convolutional neural network and dense crowd technology, applied in the field of dense crowd counting algorithms, can solve problems such as high crowd density, inaccurate counting, and low resolution
- Summary
- Abstract
- Description
- Claims
- Application Information
AI Technical Summary
Problems solved by technology
Method used
Image
Examples
Embodiment Construction
[0022] Such as figure 1 As shown, a dense crowd counting algorithm based on a cascaded high-resolution convolutional neural network includes: using the geometric adaptive Gaussian response technology GAK to estimate the scale of a single head in a dense crowd map, and then generating a supervised prediction density map D p ; Use the primary high-resolution feature extraction network HRNet to extract the high-resolution features of the input image; use the high-resolution features to predict the density image D corresponding to the primary dense crowd p1 ;Based on the primary high-resolution feature extraction network, construct a cascaded high-resolution feature extraction network CHRNet to extract the second-level high-resolution features; adopt the regional loss weighting method, and use MSE and counting error two loss functions to optimize network parameters; use The second level of high-resolution features predicts the final dense crowd density map D p2 ; using the final ...
PUM
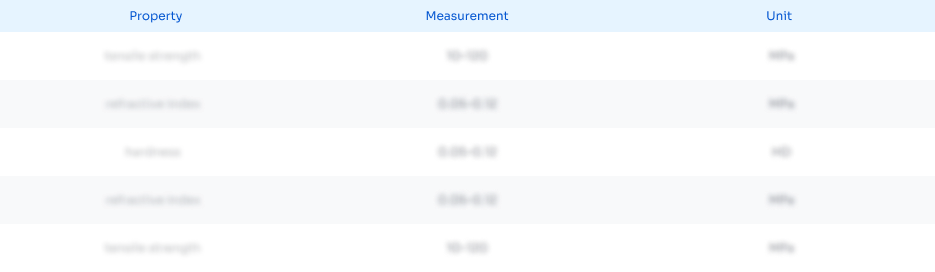
Abstract
Description
Claims
Application Information
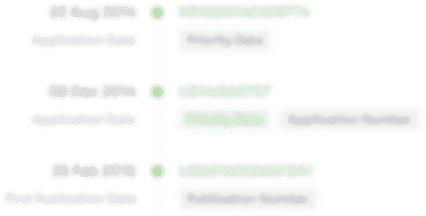
- R&D Engineer
- R&D Manager
- IP Professional
- Industry Leading Data Capabilities
- Powerful AI technology
- Patent DNA Extraction
Browse by: Latest US Patents, China's latest patents, Technical Efficacy Thesaurus, Application Domain, Technology Topic.
© 2024 PatSnap. All rights reserved.Legal|Privacy policy|Modern Slavery Act Transparency Statement|Sitemap