Nonlinear hash image retrieval method based on equivalent continuous change binary discrete optimization
A discrete optimization and image retrieval technology, applied in still image data retrieval, neural learning methods, still image data query, etc., can solve problems such as large cumulative error, non-convergence of optimization, unequal continuous optimization of binary discrete optimization problems, etc. , to achieve the effect of ensuring convergence, solving the slack inequality problem, and strengthening the ability of semantic learning
- Summary
- Abstract
- Description
- Claims
- Application Information
AI Technical Summary
Problems solved by technology
Method used
Image
Examples
Embodiment Construction
[0044] In order to make the purpose, technical solution and advantages of the present invention clearer, the present invention will be further described in detail below in conjunction with specific embodiments and with reference to the accompanying drawings.
[0045] refer to figure 1 and figure 2 , a non-linear hash image retrieval method based on binary discrete optimization of equivalent continuous variation, comprising the following steps:
[0046]Step 1: Refer to figure 2 , to obtain a binary discrete optimization method for the general hash learning model based on equivalent continuous changes, the process is as follows:
[0047] Step 1.1: Construct a quantized general hash learning model, which is a binary discrete optimization problem:
[0048]
[0049] where B is the original image set The corresponding hash code set, f(B) is the objective function of the general hash learning model.
[0050] Step 1.2: Convert the binary discrete optimization problem obtain...
PUM
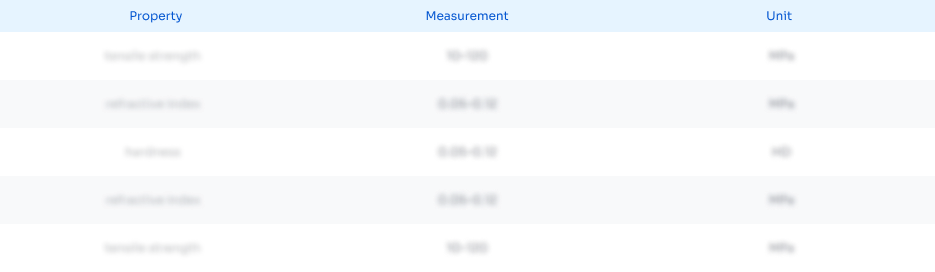
Abstract
Description
Claims
Application Information
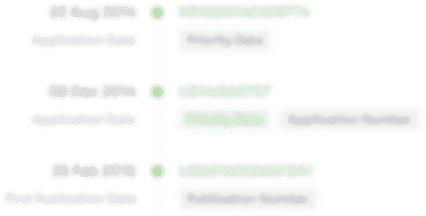
- R&D Engineer
- R&D Manager
- IP Professional
- Industry Leading Data Capabilities
- Powerful AI technology
- Patent DNA Extraction
Browse by: Latest US Patents, China's latest patents, Technical Efficacy Thesaurus, Application Domain, Technology Topic, Popular Technical Reports.
© 2024 PatSnap. All rights reserved.Legal|Privacy policy|Modern Slavery Act Transparency Statement|Sitemap|About US| Contact US: help@patsnap.com