Face recognition method and system based on anti-noise meta-learning
A face recognition and meta-learning technology, applied in the field of face recognition, can solve problems such as poor recognition effect, unstable face recognition model, and many wrong labels in large face recognition data sets, and achieve good face recognition performance. , Accurate identification, time-saving effect of training process
- Summary
- Abstract
- Description
- Claims
- Application Information
AI Technical Summary
Problems solved by technology
Method used
Image
Examples
Embodiment 1
[0050] Face recognition methods based on anti-noise meta-learning, such as figure 1 shown, including the following steps:
[0051] S1. Obtain a face dataset and its corresponding labels, where the labels include noise labels and clean labels;
[0052] S2. Preprocess the face data set: sample small batches of face data (X, Y) from the face data set, where X={x 1 ,…,x k } is k face data, Y={y 1 ,…,y k } is the corresponding noise label of each face data; for each noise label, multiple noise labels {Y′ are generated 1, …,Y′ M }, whose distribution is similar to Y, so that a set of generated noise labels Y′ m ={y' m1 ,…,y′ mk };
[0053] At the beginning of the training process, the face recognition model is not capable of face recognition. Therefore, all face data should be initialized with the same weight parameters for subsequent training.
[0054] S3. construct a face recognition model and its meta-learning model learning strategy, the input of the face recognition ...
Embodiment 2
[0069] Face recognition systems based on anti-noise meta-learning, such as figure 2 shown, including:
[0070] A data acquisition module 1 is used to acquire a face dataset and its corresponding labels, wherein the labels include noise labels and clean labels;
[0071] Data initialization module 2, for preprocessing the face data set, generating synthetic noise labels for each face data therein, and initializing the same weight parameters for each face data;
[0072] A face recognition model and its meta-learning model learning strategy building module 3 is used to construct a face recognition model and its meta-learning model learning strategy, the input of the face recognition model is the face data to be recognized, and the output of the The recognition result of the recognized face data; the meta-learning model learning strategy adopts the gradient descent algorithm to update the weight parameter of the face recognition model;
[0073] The meta-learning model training m...
PUM
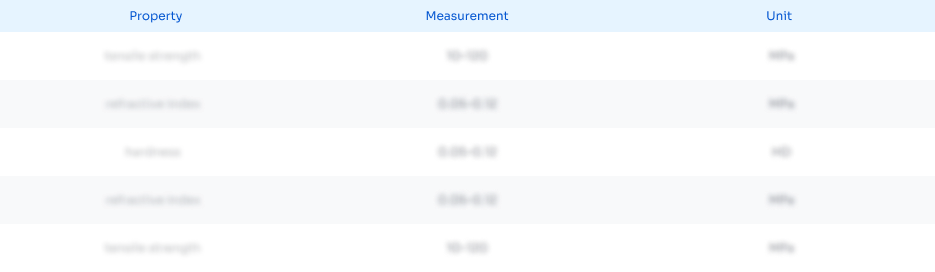
Abstract
Description
Claims
Application Information
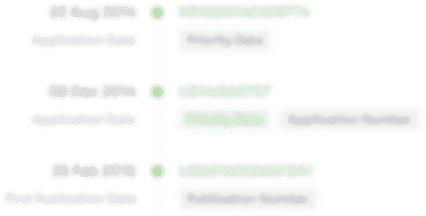
- R&D Engineer
- R&D Manager
- IP Professional
- Industry Leading Data Capabilities
- Powerful AI technology
- Patent DNA Extraction
Browse by: Latest US Patents, China's latest patents, Technical Efficacy Thesaurus, Application Domain, Technology Topic, Popular Technical Reports.
© 2024 PatSnap. All rights reserved.Legal|Privacy policy|Modern Slavery Act Transparency Statement|Sitemap|About US| Contact US: help@patsnap.com