Semi-supervised classification algorithm based on dimension weighting and view angle feature consistency
A classification algorithm and consistency technology, applied to other database clustering/classification, computing, computer components, etc., can solve problems such as ignoring relationships, ignoring local structure information of data, and affecting classification results
- Summary
- Abstract
- Description
- Claims
- Application Information
AI Technical Summary
Problems solved by technology
Method used
Image
Examples
Embodiment Construction
[0055] The present invention will be further described below in conjunction with the accompanying drawings and embodiments, and the present invention includes but not limited to the following embodiments.
[0056] Such as figure 1 As shown, the present invention provides a semi-supervised classification algorithm based on dimension weighting and view feature consistency, and its specific implementation process is as follows:
[0057] 1. Initialize the similarity matrix
[0058] Let χ={X 1 ,X 2 ,...,X V} represents a multi-view dataset, where, Represents the feature of the vth view, v=1,2,...,V, V is the number of views, n represents the number of samples, d(v) represents the dimensionality of the vth view feature.
[0059] In the Euclidean space, if the distance between two samples is closer, it means that the similarity between the two samples is higher, and they should have the same output category. Furthermore, there is complementarity and consistency among multi-vie...
PUM
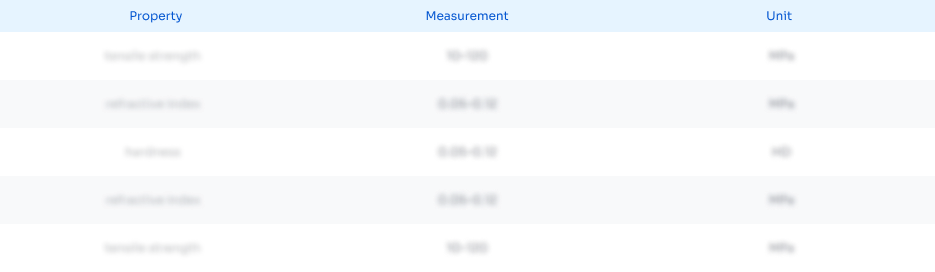
Abstract
Description
Claims
Application Information
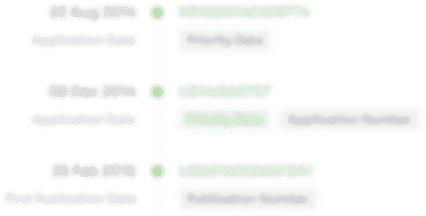
- R&D
- Intellectual Property
- Life Sciences
- Materials
- Tech Scout
- Unparalleled Data Quality
- Higher Quality Content
- 60% Fewer Hallucinations
Browse by: Latest US Patents, China's latest patents, Technical Efficacy Thesaurus, Application Domain, Technology Topic, Popular Technical Reports.
© 2025 PatSnap. All rights reserved.Legal|Privacy policy|Modern Slavery Act Transparency Statement|Sitemap|About US| Contact US: help@patsnap.com