Abnormity detection method and device for large-scale log data and storage medium
A large-scale technology for anomaly detection, applied in neural learning methods, electrical digital data processing, error detection/correction, etc. More effective, more efficient effect
- Summary
- Abstract
- Description
- Claims
- Application Information
AI Technical Summary
Problems solved by technology
Method used
Image
Examples
Embodiment 1
[0030] Embodiment 1. An anomaly detection method for large-scale log data, such as figure 1 As shown, it includes the following steps: input the selected log sequence with a set length into the pre-built machine learning prediction model, and output the conditional probability of each log template appearing at the current position; filter the log templates according to the conditional probabilities of each log template , get the set of candidate log templates;
[0031] Analyze the log to be detected to obtain its log template; judge whether the log template corresponding to the log to be detected belongs to the set of candidate log templates, if so, determine that the log is normal, and if not, judge that the log is abnormal.
[0032] After observing and researching the log sequence, it is found that in the actual environment, the number of follow-up logs of different log sequences varies greatly. Some log sequences may have a lot of logs behind them, and some may only have on...
Embodiment 2
[0044] Embodiment 2. On the basis of Embodiment 1, this embodiment provides an anomaly detection method for large-scale log data, which also includes parsing the original logs collected in advance according to the order in which task identifiers appear. to reorder. The principle of anomaly detection based on sequence prediction is that the model trained by normal logs can mine and identify the normal behavior patterns in the logs, so as to predict subsequent logs. However, since there are many concurrently executed jobs in the system, there are multiple logs of the same task (identified uniquely by session_id). The session_id of these logs is the same, but they are not consecutive in the original log. In order to get For a better training effect, the original logs need to be reordered according to the order in which task session_ids appear: that is, to arrange multiple logs generated by concurrent execution of a task together, and to sort and sort multiple logs generated by mu...
Embodiment 4
[0053] Embodiment 4, the present invention provides a large-scale log data anomaly detection device (structure such as Figure 5 shown), including log parsing module, log template candidate set determination module and log anomaly detection module:
[0054] The log analysis module is used to analyze the log to be detected to obtain its log template;
[0055] The log template candidate set determination module is used to input the selected log sequence with a set length into the pre-built machine learning prediction model, and output the conditional probability of each log template at the current position; according to the conditional probability of each log template, the log template Perform screening to obtain a set of candidate log templates;
[0056] The log anomaly detection module is used for judging whether the log template corresponding to the log to be detected by the log parsing module belongs to the set of candidate log templates, and if so, it is judged that the lo...
PUM
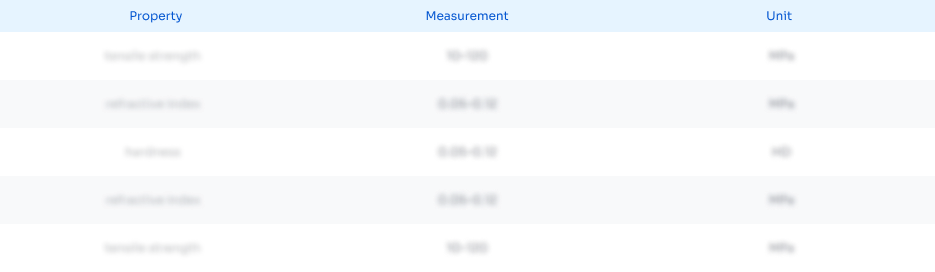
Abstract
Description
Claims
Application Information
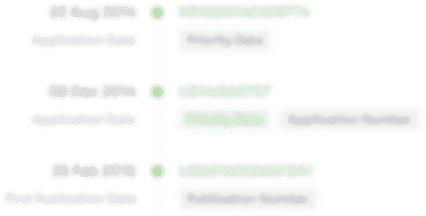
- R&D Engineer
- R&D Manager
- IP Professional
- Industry Leading Data Capabilities
- Powerful AI technology
- Patent DNA Extraction
Browse by: Latest US Patents, China's latest patents, Technical Efficacy Thesaurus, Application Domain, Technology Topic.
© 2024 PatSnap. All rights reserved.Legal|Privacy policy|Modern Slavery Act Transparency Statement|Sitemap