Fine-grained cross-media retrieval method based on multi-model network
A cross-media, fine-grained technology, applied in the fields of multimedia retrieval, fine-grained recognition, natural language processing, and computer vision, can solve the problems of media heterogeneity, small differences between classes, and large differences within classes, etc., to reduce heterogeneity Gap problem, the effect of improving accuracy
- Summary
- Abstract
- Description
- Claims
- Application Information
AI Technical Summary
Problems solved by technology
Method used
Image
Examples
Embodiment Construction
[0061] Such as figure 1 As shown, a fine-grained cross-media retrieval method based on multi-model network, including the following steps:
[0062]Step 1. Obtain the PKU FG-XMedia dataset, which is currently the only fine-grained cross-media dataset, containing 200 fine-grained categories of birds, including four media types: image, video, text and audio. And preprocess the data set to obtain cross-media data.
[0063] Specifically, the preprocessing method is as follows: for pictures and texts, no processing is required; for videos, 25 frames are equally spaced for each video as video data; for audio, short-time Fourier transform is used to obtain a spectrogram as audio data.
[0064] Step 2, respectively extracting the proprietary features of each media data, specifically including:
[0065] Using a feature extractor based on bilinear CNN to extract image and video data features, the specific process is:
[0066] Such as figure 2 As shown, two CNN networks can be regar...
PUM
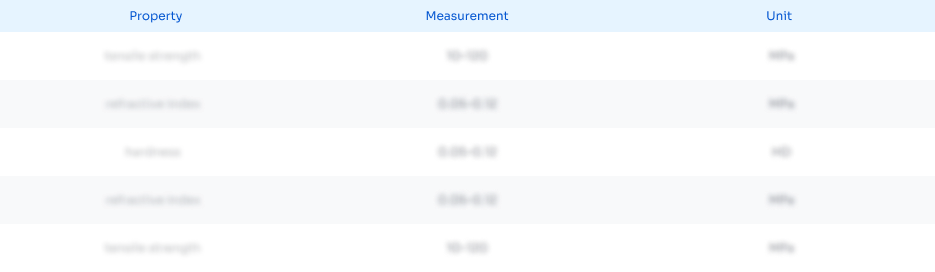
Abstract
Description
Claims
Application Information
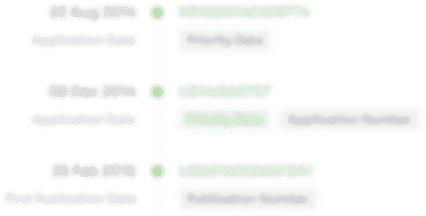
- R&D Engineer
- R&D Manager
- IP Professional
- Industry Leading Data Capabilities
- Powerful AI technology
- Patent DNA Extraction
Browse by: Latest US Patents, China's latest patents, Technical Efficacy Thesaurus, Application Domain, Technology Topic, Popular Technical Reports.
© 2024 PatSnap. All rights reserved.Legal|Privacy policy|Modern Slavery Act Transparency Statement|Sitemap|About US| Contact US: help@patsnap.com