Method and system for multi-label distribution learning in natural language processing classification model
A technology of natural language processing and classification model, applied in natural language data processing, semantic analysis, electronic digital data processing and other directions, can solve the problems of inaccurate label distribution and unfavorable model generalization ability.
- Summary
- Abstract
- Description
- Claims
- Application Information
AI Technical Summary
Problems solved by technology
Method used
Image
Examples
Embodiment Construction
[0049] In order to make the purpose, technical solution and advantages of the present application clearer, the technical solution of the present invention will be described in detail below in conjunction with the drawings and embodiments. Apparently, the described embodiments are only some of the embodiments of this application, not all of them. Based on the embodiments in the present application, all other implementation manners obtained by persons of ordinary skill in the art without creative efforts fall within the protection scope of the present application.
[0050] In one embodiment, the present invention provides a method for multi-label distribution learning in a natural language processing classification model, such as figure 1 shown, including the following steps:
[0051] Get training samples;
[0052] Calculate the label vector of each label and the sample vector of each sample according to the data of all samples;
[0053] Calculate the correlation between each...
PUM
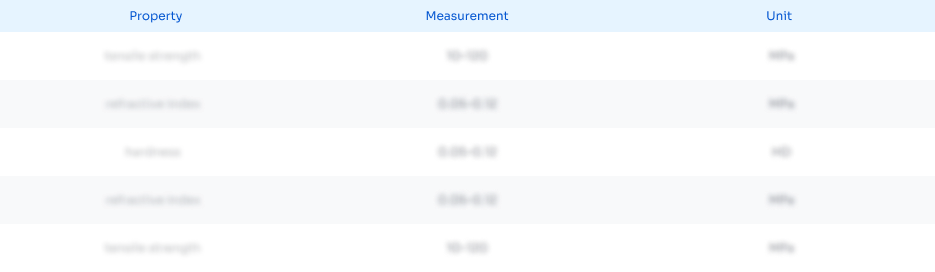
Abstract
Description
Claims
Application Information
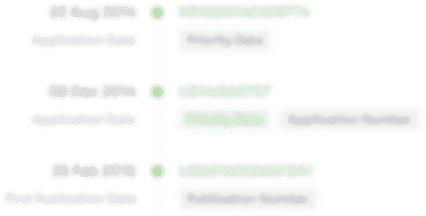
- R&D
- Intellectual Property
- Life Sciences
- Materials
- Tech Scout
- Unparalleled Data Quality
- Higher Quality Content
- 60% Fewer Hallucinations
Browse by: Latest US Patents, China's latest patents, Technical Efficacy Thesaurus, Application Domain, Technology Topic, Popular Technical Reports.
© 2025 PatSnap. All rights reserved.Legal|Privacy policy|Modern Slavery Act Transparency Statement|Sitemap|About US| Contact US: help@patsnap.com