Small sample remote sensing ground object classification method and system based on feature generation network
A technology for classifying ground objects and types of ground objects, applied in the field of remote sensing intelligent recognition, which can solve problems such as impracticality, separate training, and difficulty in training data sets.
- Summary
- Abstract
- Description
- Claims
- Application Information
AI Technical Summary
Problems solved by technology
Method used
Image
Examples
Embodiment 1
[0066] Such as figure 1 As shown, a kind of small-sample remote sensing object classification method proposed by the present invention includes:
[0067] S1: Divide the remote sensing surface object classification dataset into source dataset D based on the object category source and the target dataset D target . Such as image 3 As shown in , it is divided into source category and test category according to the object category, and it is guaranteed that there is no overlap between the two object categories. Further according to the source category and the target category, the remote sensing ground object classification dataset is divided into source dataset D source , target data set D target , and ensure that the source data set D source The number of samples for each category is generally not less than 500. Among them, the types of ground objects in the source data set include but are not limited to: buildings, roads, and grasslands, which are used for model training;...
Embodiment 2
[0088] In order to realize the above method, the present invention also provides a small-sample remote sensing object classification system, such as Figure 5 shown, including:
[0089] Dataset building block: used to divide remote sensing object classification datasets into source datasets D based on object categories source and the target dataset D target ;
[0090] The model training module is used in the source data set D source On the feature extractor F and the generation network G are trained;
[0091] Generate a feature set module for the target dataset D based on target , using the feature extractor F and the generation network G to perform feature generation to obtain the generated feature sets corresponding to each object type;
[0092] The classification module is used to use the feature extractor F to perform feature extraction on the test feature image x, and perform similarity measurement between the test sample features and the generated sample sets of var...
PUM
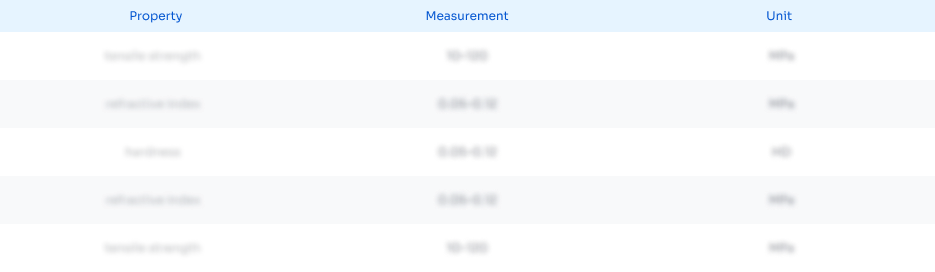
Abstract
Description
Claims
Application Information
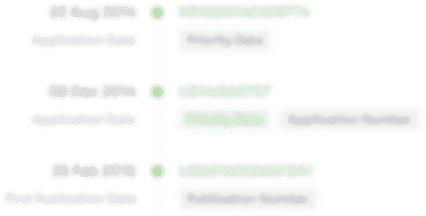
- R&D Engineer
- R&D Manager
- IP Professional
- Industry Leading Data Capabilities
- Powerful AI technology
- Patent DNA Extraction
Browse by: Latest US Patents, China's latest patents, Technical Efficacy Thesaurus, Application Domain, Technology Topic.
© 2024 PatSnap. All rights reserved.Legal|Privacy policy|Modern Slavery Act Transparency Statement|Sitemap