Image defogging method for haze concentration of adaptive neural network based on end-to-end
A haze concentration, neural network technology, applied in the field of image dehazing based on end-to-end haze concentration adaptive neural network, can solve the problems of complex, prior information invalidation calculation and so on
- Summary
- Abstract
- Description
- Claims
- Application Information
AI Technical Summary
Problems solved by technology
Method used
Image
Examples
Embodiment Construction
[0043] see figure 1 , figure 2 , image 3 and Figure 4 , an end-to-end haze concentration-based self-adaptive neural network image defogging method disclosed by the present invention comprises the following steps:
[0044]S1. Constructing an image dehazing model, wherein the image dehazing model includes a pyramid feature extractor, a feature enhancement module and a multi-scale feature attention module;
[0045] The specific process is as figure 2 As shown, the image dehazing model is constructed. Image dehazing models include pyramidal feature extractors such as figure 2 shown), feature enhancement modules (such as figure 2 shown) and a multi-scale feature attention module (such as figure 2 shown);
[0046] S2. Obtain foggy image data, use the pyramid feature extractor to extract the feature maps of the four different layers of the fog image through the convolutional neural network, and fuse information of different scales to generate useful information; mainly...
PUM
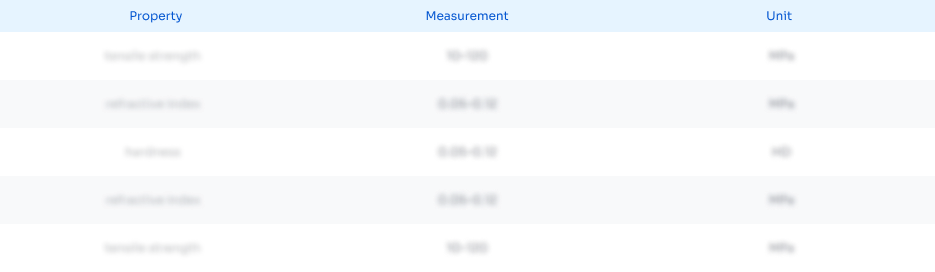
Abstract
Description
Claims
Application Information
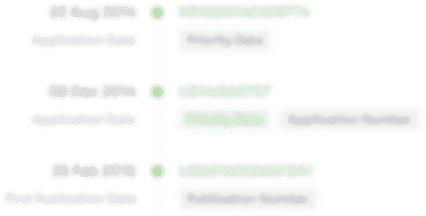
- R&D
- Intellectual Property
- Life Sciences
- Materials
- Tech Scout
- Unparalleled Data Quality
- Higher Quality Content
- 60% Fewer Hallucinations
Browse by: Latest US Patents, China's latest patents, Technical Efficacy Thesaurus, Application Domain, Technology Topic, Popular Technical Reports.
© 2025 PatSnap. All rights reserved.Legal|Privacy policy|Modern Slavery Act Transparency Statement|Sitemap|About US| Contact US: help@patsnap.com