Citation difference matching method, device and storage medium based on mg-lstm
A matching method and citation technology, applied in neural learning methods, text database query, unstructured text data retrieval and other directions, can solve the problems of low discovery efficiency, difficult to guarantee accuracy, large workload, etc. The effect of ensuring the screening accuracy
- Summary
- Abstract
- Description
- Claims
- Application Information
AI Technical Summary
Problems solved by technology
Method used
Image
Examples
Embodiment 1
[0059] In order to realize the purpose of the present invention, it is first necessary to build and train a citation difference recognition model, and the specific process is as follows.
[0060] Citations include metadata such as titles, authors, publishers, etc. Since different citations are composed of different metadata and have different text characteristics, this embodiment uses two types of word embedding and character embedding, and the corresponding The title, author, and publisher are segmented at the granularity of words or characters, and then their sequences are mapped to a low-dimensional vector space. In this embodiment, the title metadata is taken as an example for specific description. First, the title metadata of the citation to be screened and the credible citation with converted to caption embedding vectors, respectively with Caption embedding vector with Form the title embedding vector pair, with The elements in are the embedded representati...
Embodiment 2
[0094] This embodiment provides a citation difference matching device based on MG-LSTM, including:
[0095] Data acquisition module: used to acquire the title, author and publisher metadata of citations to be screened and credible citations;
[0096] Granular Segmentation Module: It is used to divide and convert the title, author, and publisher metadata of the citations to be screened and credible citations into title embedding vector pairs, author embedding vector pairs, and publishing house embedding vector pairs using words and characters as the segmentation granularity. ;
[0097] Embedded vector weighting module: used to learn the weights of the title embedding vector pair, author embedding vector pair, and publisher embedding vector pair respectively based on the attention mechanism, and update the title embedding vector pair, author embedding vector pair, and publishing house embedding vector pair based on the corresponding weights right;
[0098] Citation difference ...
Embodiment 3
[0106] This embodiment provides a computer-readable storage medium, which stores a computer program, and the computer program is suitable for being loaded by a processor and executing the MG-LSTM-based citation difference matching method as described in Embodiment 1.
[0107] Those skilled in the art should understand that the embodiments of the present application may be provided as methods, systems, or computer program products. Accordingly, the present application may take the form of an entirely hardware embodiment, an entirely software embodiment, or an embodiment combining software and hardware aspects. Furthermore, the present application may take the form of a computer program product embodied on one or more computer-usable storage media (including but not limited to disk storage, CD-ROM, optical storage, etc.) having computer-usable program code embodied therein.
PUM
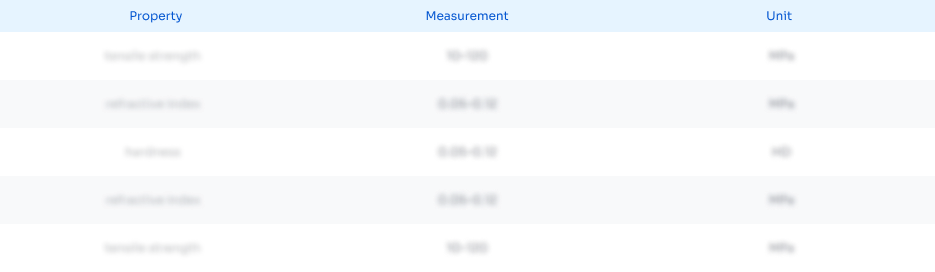
Abstract
Description
Claims
Application Information
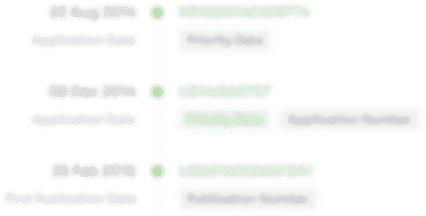
- R&D
- Intellectual Property
- Life Sciences
- Materials
- Tech Scout
- Unparalleled Data Quality
- Higher Quality Content
- 60% Fewer Hallucinations
Browse by: Latest US Patents, China's latest patents, Technical Efficacy Thesaurus, Application Domain, Technology Topic, Popular Technical Reports.
© 2025 PatSnap. All rights reserved.Legal|Privacy policy|Modern Slavery Act Transparency Statement|Sitemap|About US| Contact US: help@patsnap.com