Rocket health degree evaluation method based on long-short-term memory auto-encoder
A long-short-term memory and autoencoder technology, which is applied in neural architecture, biological neural network model, design optimization/simulation, etc., can solve the internal connection of dynamic response engines, engine process differences cannot be well matched, and engine operating conditions Diagnose problems such as low reliability of results, achieve the effect of troubleshooting measurement faults and realizing health evaluation
- Summary
- Abstract
- Description
- Claims
- Application Information
AI Technical Summary
Problems solved by technology
Method used
Image
Examples
Embodiment 1
[0050] A rocket health assessment method based on long short-term memory autoencoder. This method takes advantage of the long-short-term memory unit to process time-series data, combines the self-encoder for unsupervised learning, automatically extracts the features of the original data during the normal startup process of the rocket, restores them to realize the abnormal monitoring of the rocket, and combines the health degree calculation function. health estimate. Specifically include the following steps:
[0051] 1. Preprocess multiple data samples X of the raw data of each sensor in the rocket and obtain and keep the preprocessing parameter X max ,X min .
[0052] The preprocessing step is divided into two steps: normalization and data format modification.
[0053] The standardization adopts the min-max standardization method, namely:
[0054]
[0055] Where x' is the data sample after normalization, x is the data sample before normalization, and x min is the smal...
Embodiment 2
[0067] Such as figure 1 As shown, this embodiment relates to a rocket health evaluation system based on long short-term memory autoencoder, including: a data input module, a sample training module, and a data monitoring module. Among them: the data input module is used to input the key raw data of the rocket engine and initially change the data format to meet the input requirements of the long-short-term memory unit; the sample training module uses the sample data to train the model, updates the parameters and saves the final trained model parameters; the data The detection module uses the trained model to monitor the data in real time, draw the data curve, and display the real-time health of the data.
[0068] The raw data is engine performance data.
[0069] Such as figure 2 with image 3 As shown, this embodiment relates to a method for diagnosing the health of a launch vehicle engine based on the above system, which specifically includes the following steps:
[0070] ...
PUM
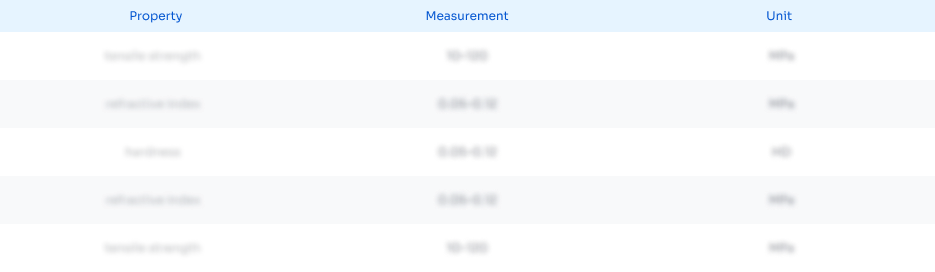
Abstract
Description
Claims
Application Information
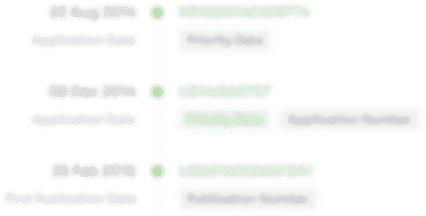
- R&D Engineer
- R&D Manager
- IP Professional
- Industry Leading Data Capabilities
- Powerful AI technology
- Patent DNA Extraction
Browse by: Latest US Patents, China's latest patents, Technical Efficacy Thesaurus, Application Domain, Technology Topic, Popular Technical Reports.
© 2024 PatSnap. All rights reserved.Legal|Privacy policy|Modern Slavery Act Transparency Statement|Sitemap|About US| Contact US: help@patsnap.com