Entity alignment method in four-insurance-one-fund domain knowledge graphs
A technology of knowledge graph and domain knowledge, applied in neural learning methods, finance, instruments, etc., can solve problems such as poor versatility, limited alignment quality, and ignoring word order information
- Summary
- Abstract
- Description
- Claims
- Application Information
AI Technical Summary
Problems solved by technology
Method used
Image
Examples
Embodiment 1
[0114] The present invention provides a knowledge map entity alignment method suitable for the field of four insurances and one fund, which can effectively solve the problem that existing methods cannot capture equivalent information from full names and abbreviations due to inconsistencies in entity names. The invention includes (1) building a knowledge map for alignment (2) dividing the original data into relational triples and attribute triplets (3) automatically marking equivalent entities for training according to entity names (4) setting attributes Threshold to filter attribute triplets (5) Train knowledge map joint embedding representation (6) Calculate LCS similarity of entities (7) Select candidate entity pairs, and disambiguate entity pairs that need to be disambiguated; specifically include the following steps :
[0115] Step 1: Input the relation triplet and attribute triplet of the knowledge graphs S and T to be aligned, and convert the data into n-triple format; ...
Embodiment 2
[0152] The present invention discovers equivalent entities from two heterogeneous knowledge graphs, and the specific process is as follows figure 1 As shown, the corpus used is the four insurances and one housing fund knowledge map and the Chinese knowledge map cn-dbpedia constructed by applying entity recognition and relationship classification technology from the text of policies and regulations. This invention will take this as an example to introduce the specific implementation of entity alignment .
[0153] Step 1: Acquisition and normalization of knowledge map data:
[0154] Step 1.1: Input the relational triples and attribute triplets of the knowledge graphs S and T to be aligned, and convert the source data into n-triple format, where each row contains a triplet, between the head entity, relation, and tail entity Use the '\t' symbol to separate. Save to rel_triples_1, rel_triples_2, attr_triples_1, attr_triples_2 four files;
[0155] Step 1.2: Mark the entities with...
PUM
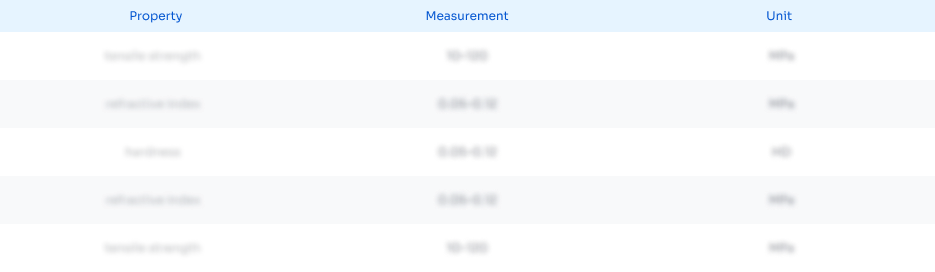
Abstract
Description
Claims
Application Information
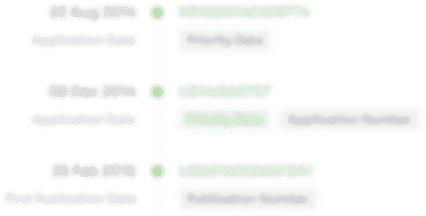
- R&D
- Intellectual Property
- Life Sciences
- Materials
- Tech Scout
- Unparalleled Data Quality
- Higher Quality Content
- 60% Fewer Hallucinations
Browse by: Latest US Patents, China's latest patents, Technical Efficacy Thesaurus, Application Domain, Technology Topic, Popular Technical Reports.
© 2025 PatSnap. All rights reserved.Legal|Privacy policy|Modern Slavery Act Transparency Statement|Sitemap|About US| Contact US: help@patsnap.com