Infectious disease collaborative prediction method based on multi-source big data deep learning, and robot
An infectious disease and data technology, applied in the field of artificial intelligence, can solve problems such as insufficient data and obstacles, and achieve the effect of improving the accuracy rate and eliminating the cost of collection
- Summary
- Abstract
- Description
- Claims
- Application Information
AI Technical Summary
Problems solved by technology
Method used
Image
Examples
Embodiment Construction
[0058] The technical solutions in the embodiments of the present invention will be described in detail below in conjunction with the embodiments of the present invention.
[0059] Basic embodiment of the invention
[0060] One embodiment of the present invention provides an artificial intelligence method, such as figure 1 As shown, the method includes: a target infectious disease determination step; an infectious disease prediction model initialization step; an optimal infectious disease prediction model construction step; an optimal infectious disease prediction model use step. Technical effect: the method obtains infectious disease prediction models in different regions through different combinations of multi-source input data training, and calculates the accuracy of different infectious disease prediction models to evaluate the credibility of the model, and then selects the most reliable As the best model in this area, the model can give its credible error range, so that i...
PUM
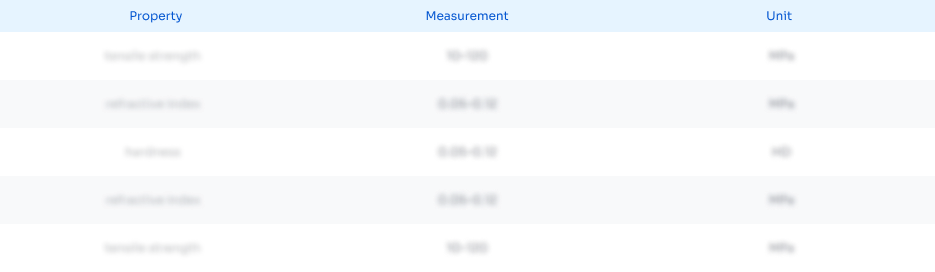
Abstract
Description
Claims
Application Information
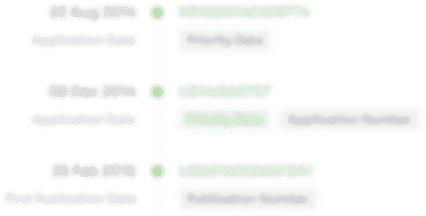
- R&D
- Intellectual Property
- Life Sciences
- Materials
- Tech Scout
- Unparalleled Data Quality
- Higher Quality Content
- 60% Fewer Hallucinations
Browse by: Latest US Patents, China's latest patents, Technical Efficacy Thesaurus, Application Domain, Technology Topic, Popular Technical Reports.
© 2025 PatSnap. All rights reserved.Legal|Privacy policy|Modern Slavery Act Transparency Statement|Sitemap|About US| Contact US: help@patsnap.com