An Image Recognition Method Based on Improved Focal Loss Function
A loss function and image recognition technology, which is applied in the field of multi-label image recognition, can solve the problems of not fully utilizing all samples, lack of partial sample data, and huge calculation amount, so as to reduce the amount of calculation, increase the loss value, and reduce the loss value. Effect
- Summary
- Abstract
- Description
- Claims
- Application Information
AI Technical Summary
Problems solved by technology
Method used
Image
Examples
Embodiment Construction
[0028] In order to illustrate the technical solution of the present invention more clearly, the technical solution of the present invention is further described in detail below in conjunction with the accompanying drawings:
[0029] The present invention proposes an image recognition method based on the improved Focal loss function, such as figure 1 shown, including the following steps:
[0030] Step 1: First divide the samples into training set, test set and remaining sample set, and then use the training set to train the convolutional neural network model based on the improved Focal loss function.
[0031] Train an improved model M based on Focal loss, such as figure 2As shown, the convolutional neural network model has five hierarchical structures: input layer, convolutional layer, activation layer, pooling layer, and fully connected layer. In this convolutional neural network structure, the input layer inputs image information, and the size of each image is 128*128. Th...
PUM
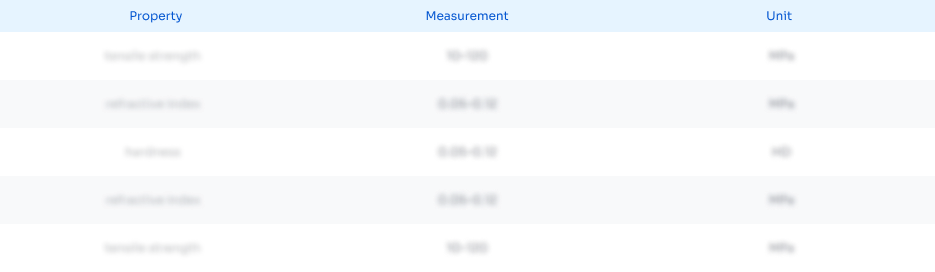
Abstract
Description
Claims
Application Information
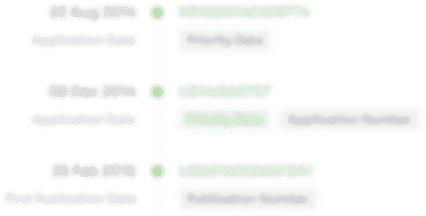
- R&D
- Intellectual Property
- Life Sciences
- Materials
- Tech Scout
- Unparalleled Data Quality
- Higher Quality Content
- 60% Fewer Hallucinations
Browse by: Latest US Patents, China's latest patents, Technical Efficacy Thesaurus, Application Domain, Technology Topic, Popular Technical Reports.
© 2025 PatSnap. All rights reserved.Legal|Privacy policy|Modern Slavery Act Transparency Statement|Sitemap|About US| Contact US: help@patsnap.com