Remote sensing image semantic segmentation method based on self-supervised contrast learning
A technology for semantic segmentation and remote sensing images, applied in neural learning methods, instruments, biological neural network models, etc., can solve problems such as category impurity semantic segmentation tasks, and achieve good results
- Summary
- Abstract
- Description
- Claims
- Application Information
AI Technical Summary
Problems solved by technology
Method used
Image
Examples
Embodiment Construction
[0053] Next, the technical solutions in the embodiments of the present invention will be apparent from the embodiment of the present invention, and it is clearly described, and it is understood that the described embodiments are merely embodiments of the present invention, not all of the embodiments.
[0054] Such as figure 1 As shown, a remote sensing image semantic segmentation method based on self-supervision comparison learning, including the following steps:
[0055] Step 1, build the DeepLab V3 + network model;
[0056] Step 2, the encoder of the network model is pre-trained with no label data;
[0057] Step 3, after the pre-training is completed, the network model has a supervisory semantics segmentation training in the labeling sample;
[0058] Step 4, use the network model with the supervisor semantic segmentation training to semantically segment the remote sensing image.
[0059] Comparative learning is to construct a characterization by learning two things, and its core...
PUM
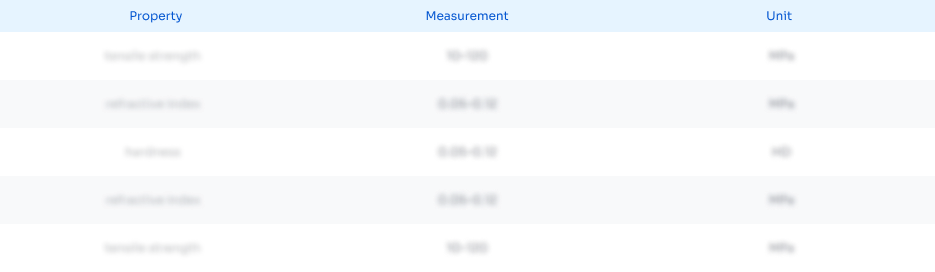
Abstract
Description
Claims
Application Information
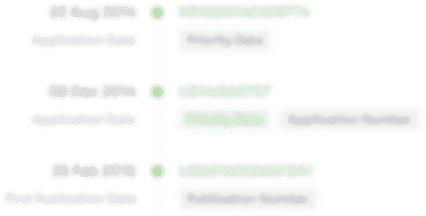
- R&D Engineer
- R&D Manager
- IP Professional
- Industry Leading Data Capabilities
- Powerful AI technology
- Patent DNA Extraction
Browse by: Latest US Patents, China's latest patents, Technical Efficacy Thesaurus, Application Domain, Technology Topic, Popular Technical Reports.
© 2024 PatSnap. All rights reserved.Legal|Privacy policy|Modern Slavery Act Transparency Statement|Sitemap|About US| Contact US: help@patsnap.com